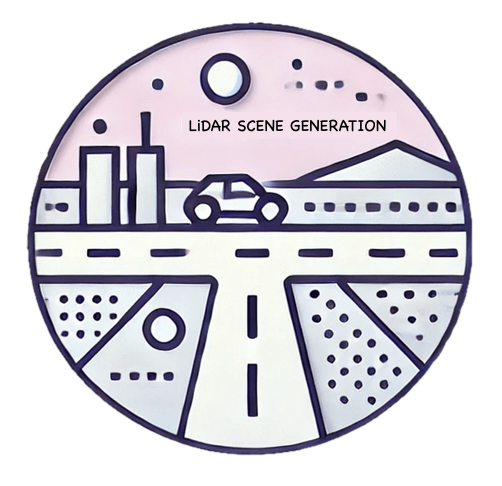
DynamicCity: Large-Scale 4D Occupancy Generation from Dynamic Scenes
ICLR 2025 Spotlight
Abstract
Urban scene generation has been developing rapidly recently. However, existing methods primarily focus on generating static and single-frame scenes, overlooking the inherently dynamic nature of real-world driving environments. In this work, we introduce DynamicCity, a novel 4D occupancy generation framework capable of generating large-scale, high-quality dynamic 4D scenes with semantics. DynamicCity mainly consists of two key models:
1. A VAE model for learning HexPlane as the compact 4D representation. Instead of using naive averaging operations, DynamicCity employs a novel Projection Module to effectively compress 4D features into six 2D feature maps for HexPlane construction, which significantly enhances HexPlane fitting quality (up to 12.56 mIoU gain). Furthermore, we utilize an Expansion & Squeeze Strategy to reconstruct 3D feature volumes in parallel, which improves both network training efficiency and reconstruction accuracy than naively querying each 3D point (up to 7.05 mIoU gain, 2.06x training speedup, and 70.84% memory reduction).
2. A DiT-based diffusion model for HexPlane generation. To make HexPlane feasible for DiT generation, a Padded Rollout Operation is proposed to reorganize all six feature planes of the HexPlane as a squared 2D feature map. In particular, various conditions could be introduced in the diffusion or sampling process, supporting versatile 4D generation applications, such as trajectory- and command-driven generation, inpainting, and layout-conditioned generation.
Extensive experiments on the CarlaSC and Waymo datasets demonstrate that DynamicCity significantly outperforms existing state-of-the-art 4D occupancy generation methods across multiple metrics. The code and models have been released to facilitate future research.
Method
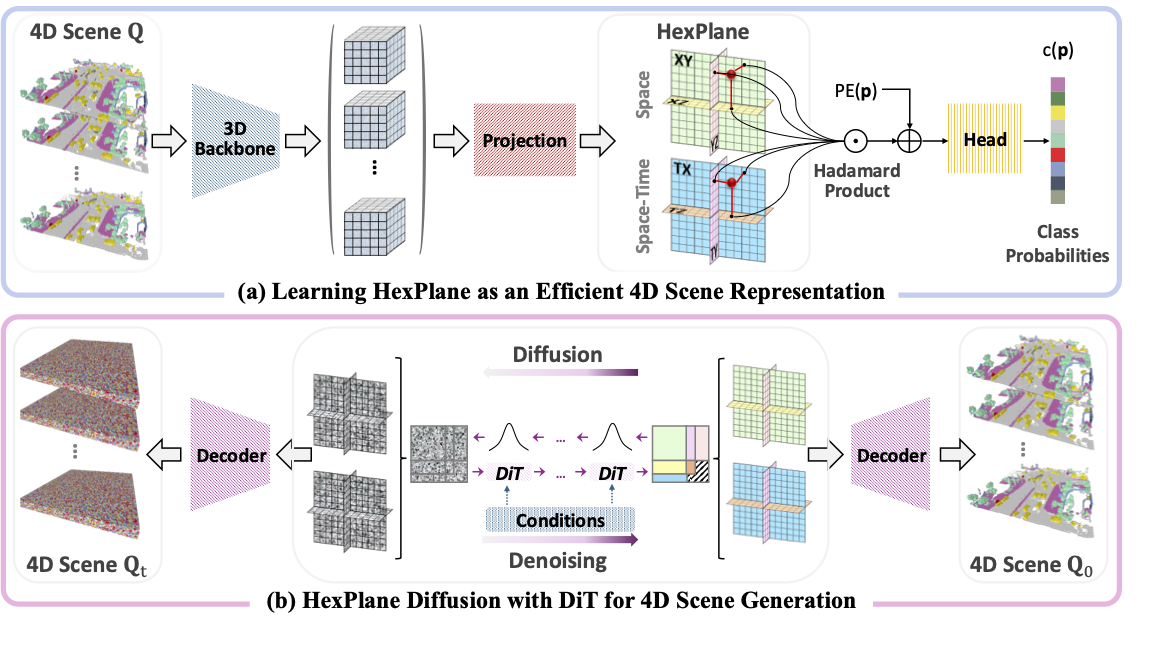
Our DynamicCity framework consists of two key procedures: (a) Encoding HexPlane with an VAE architecture, and (b) 4D Scene Generation with HexPlane DiT.
Dynamic Scene Generation Results
1. Unconditional Generation
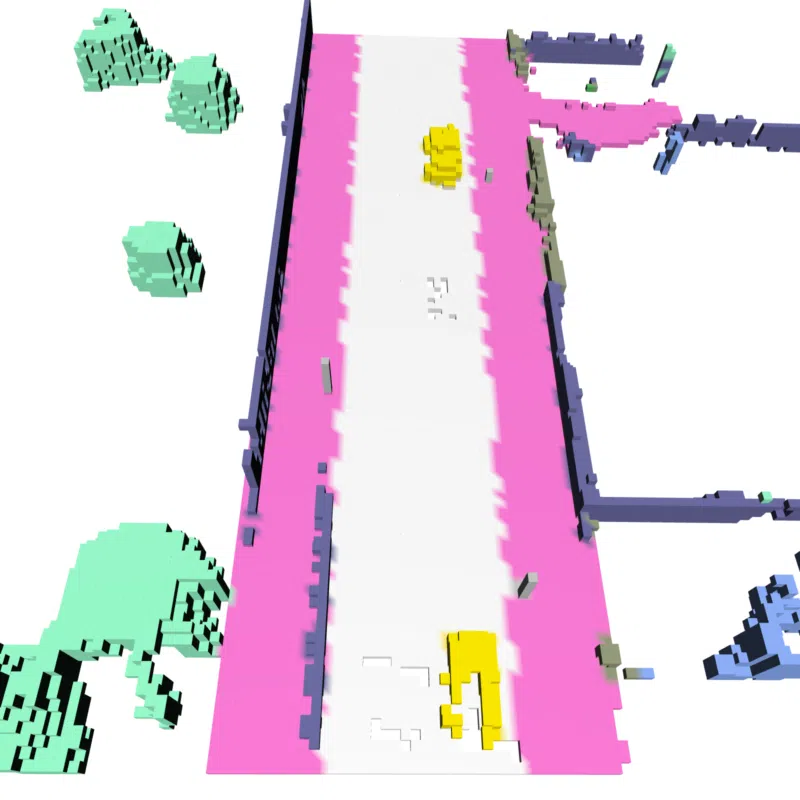
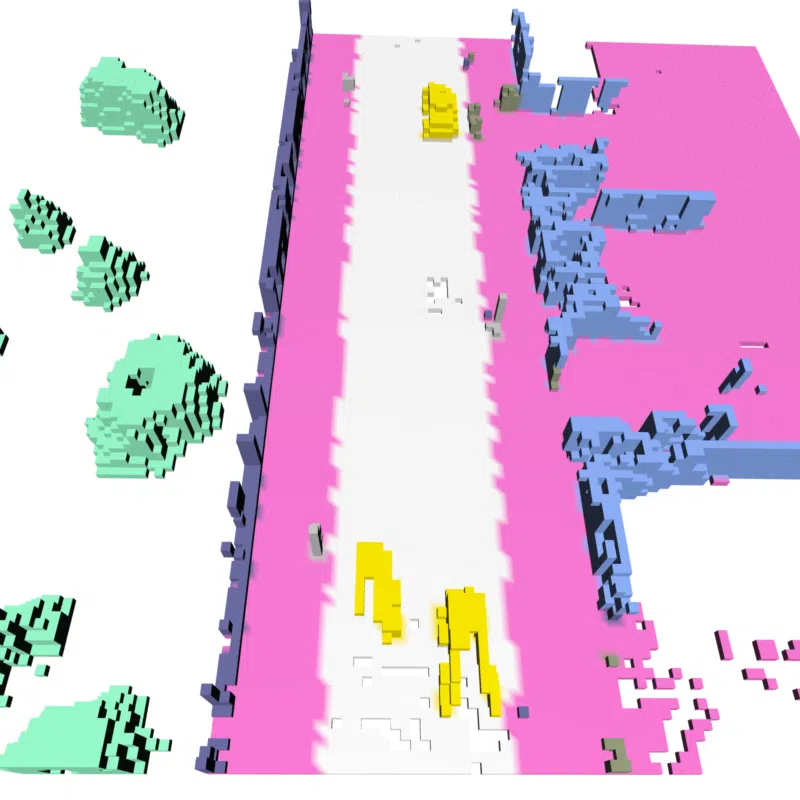
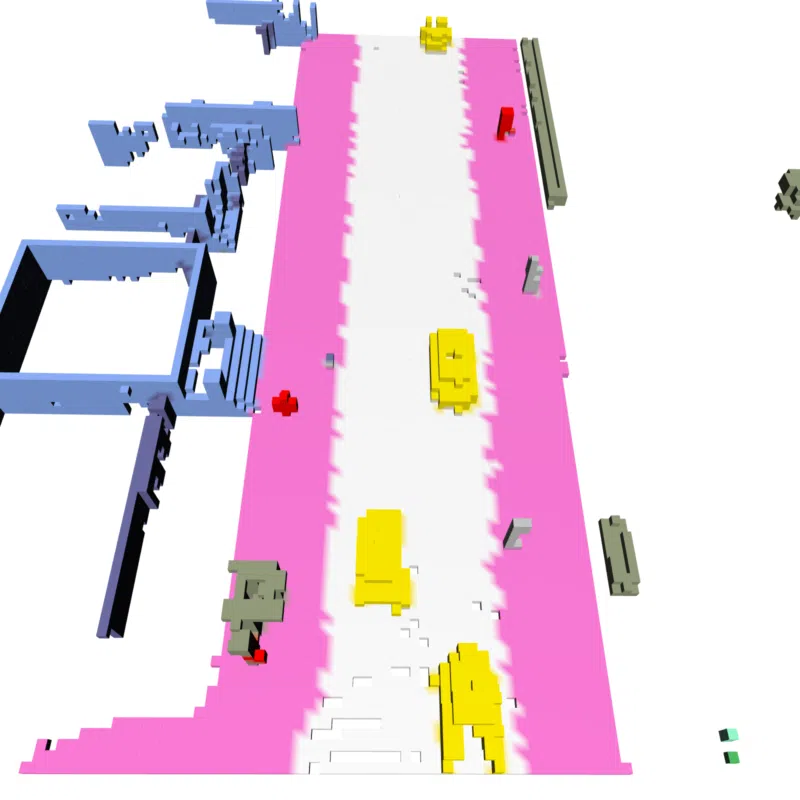
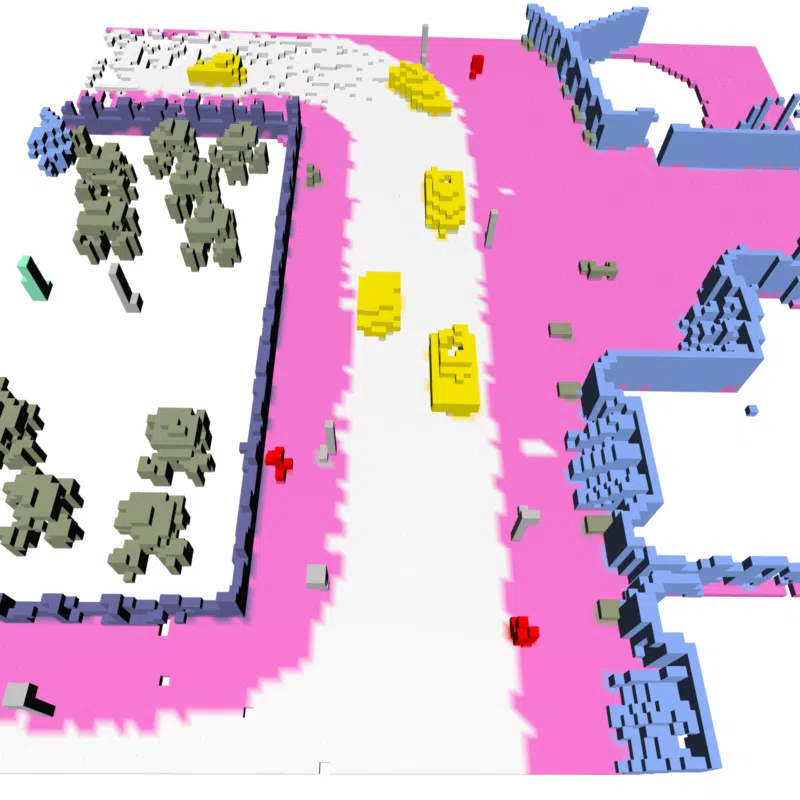
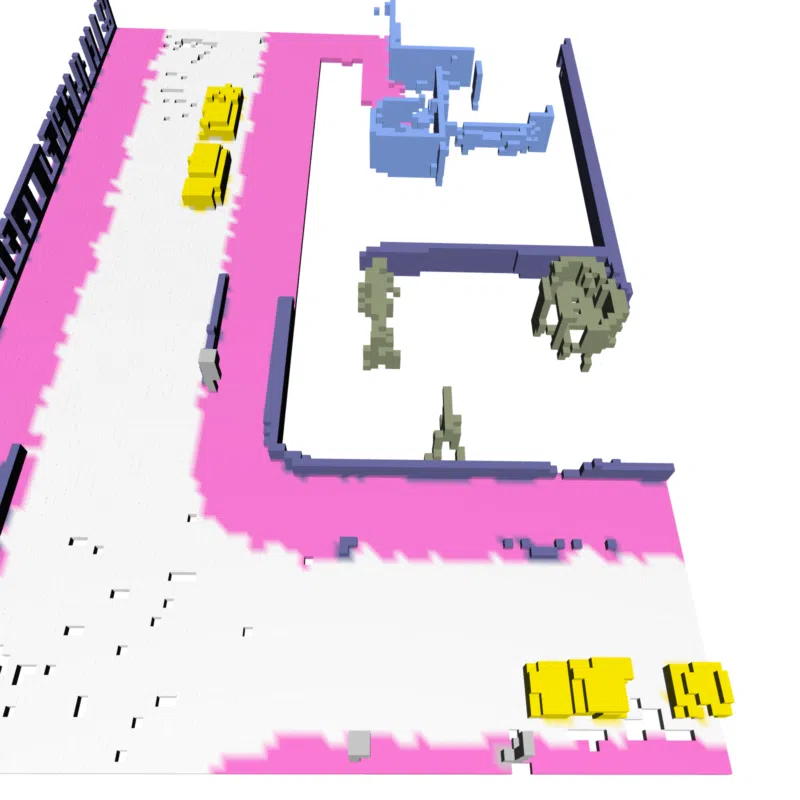
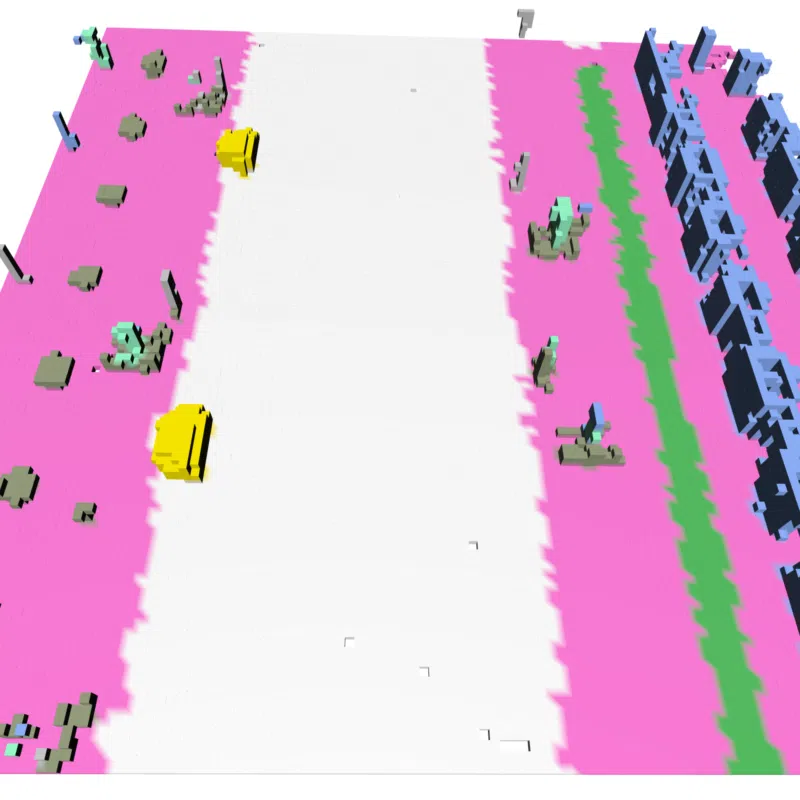
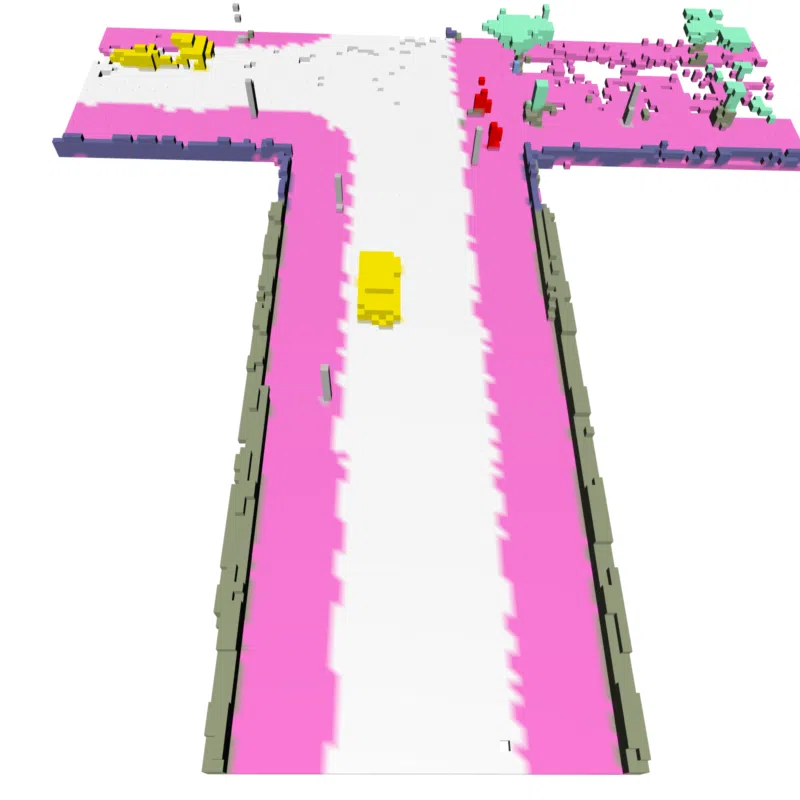
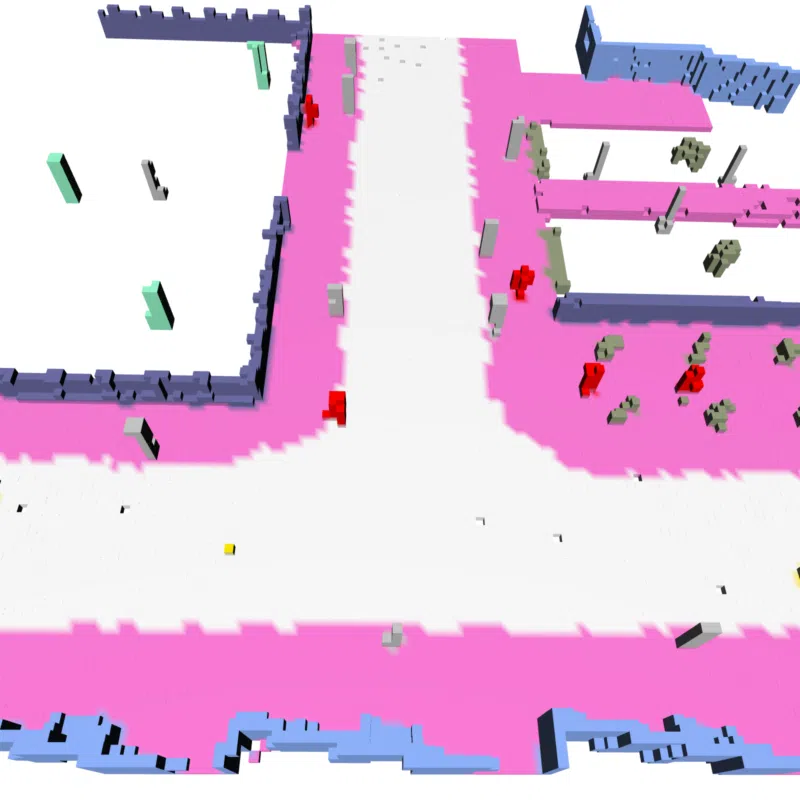
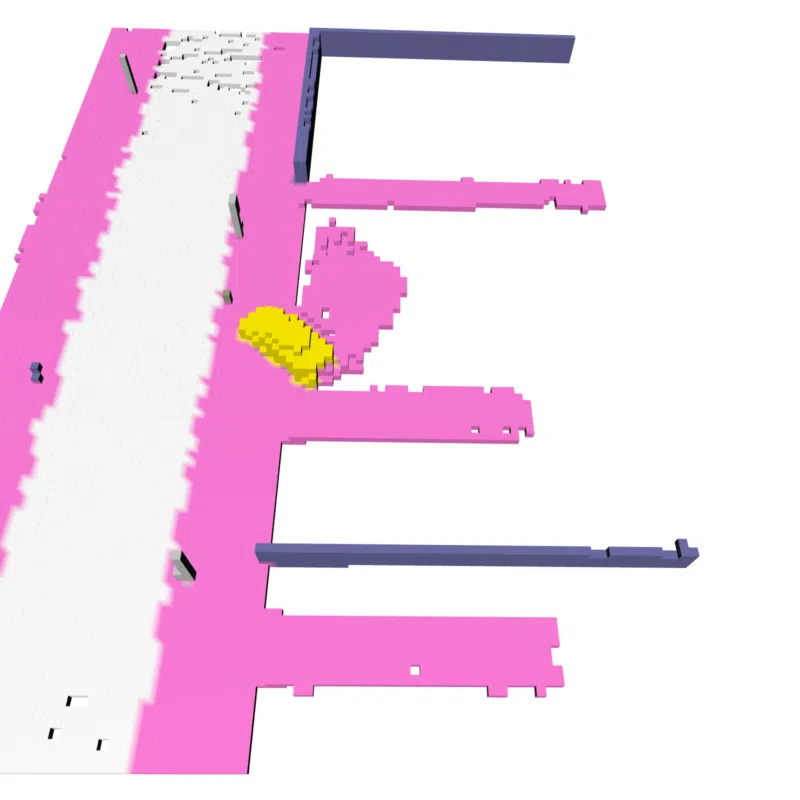
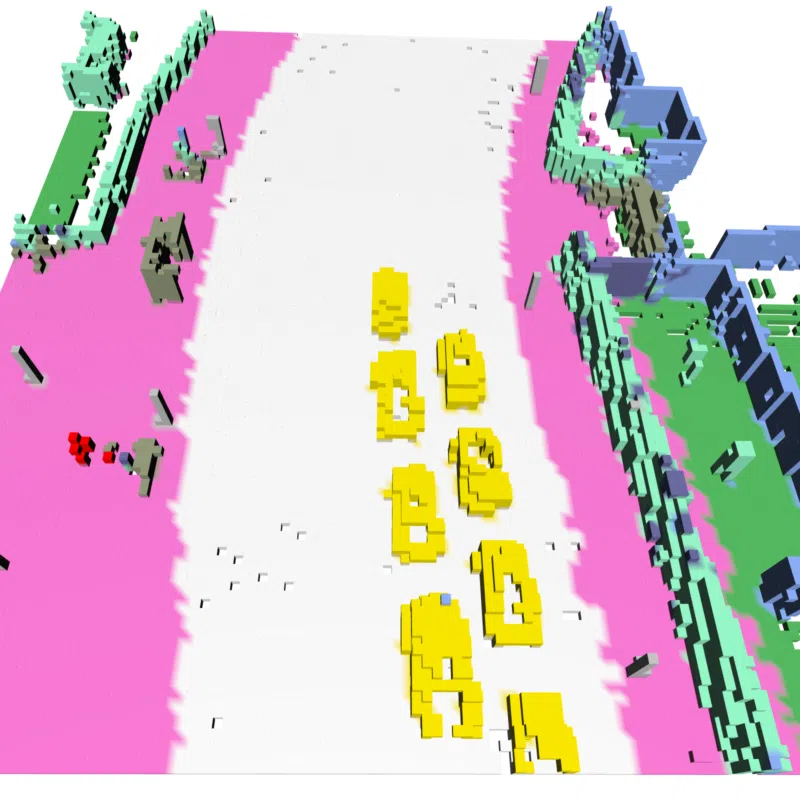
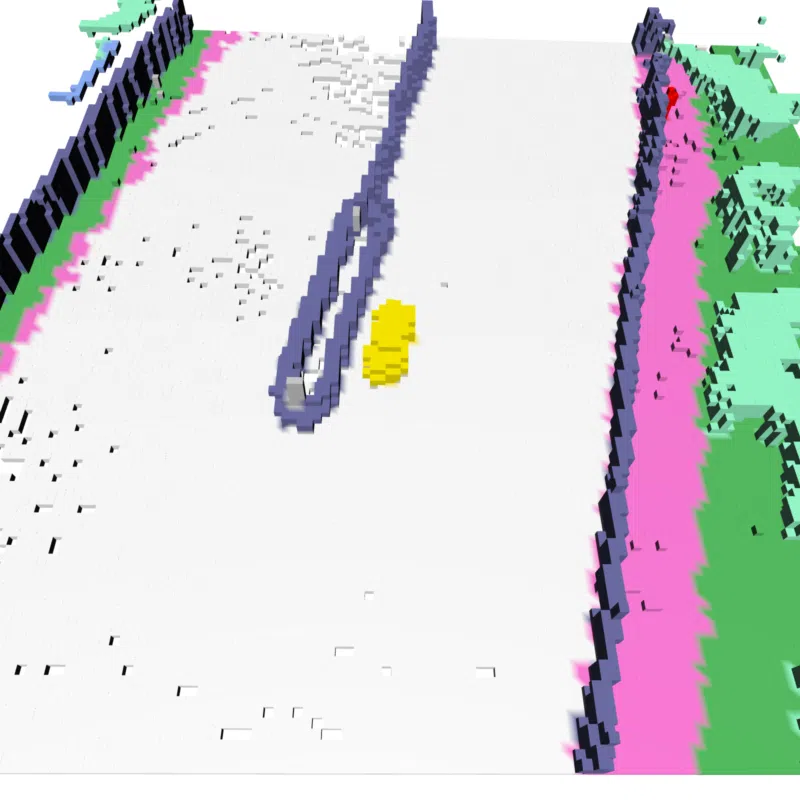
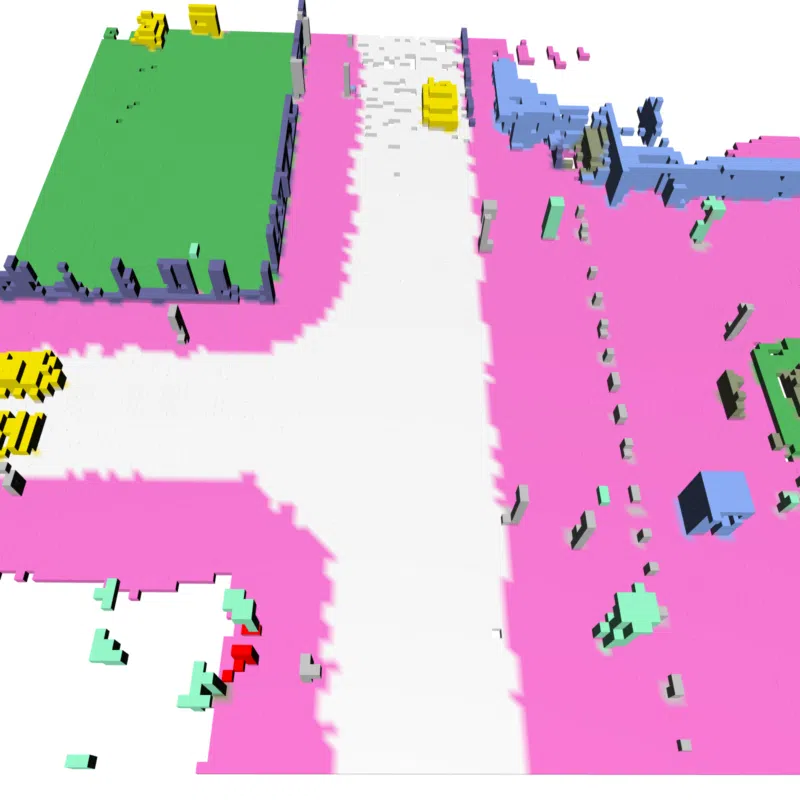
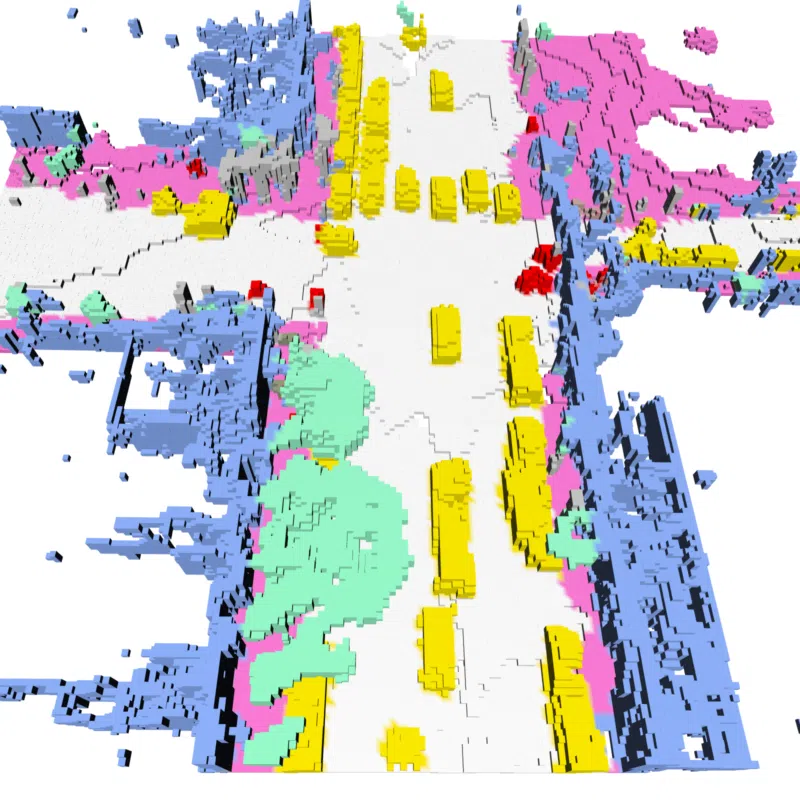
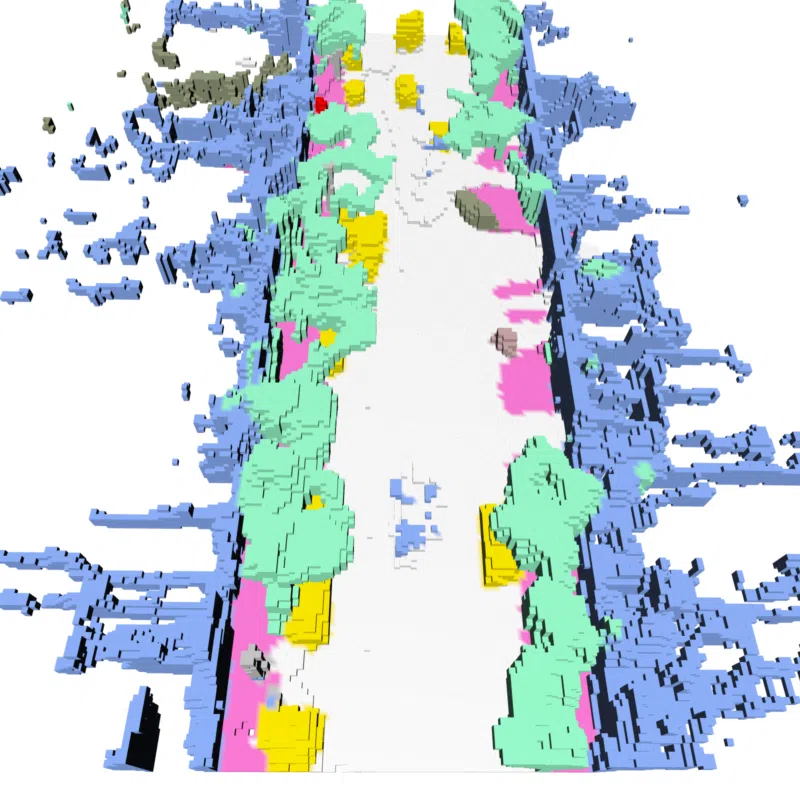
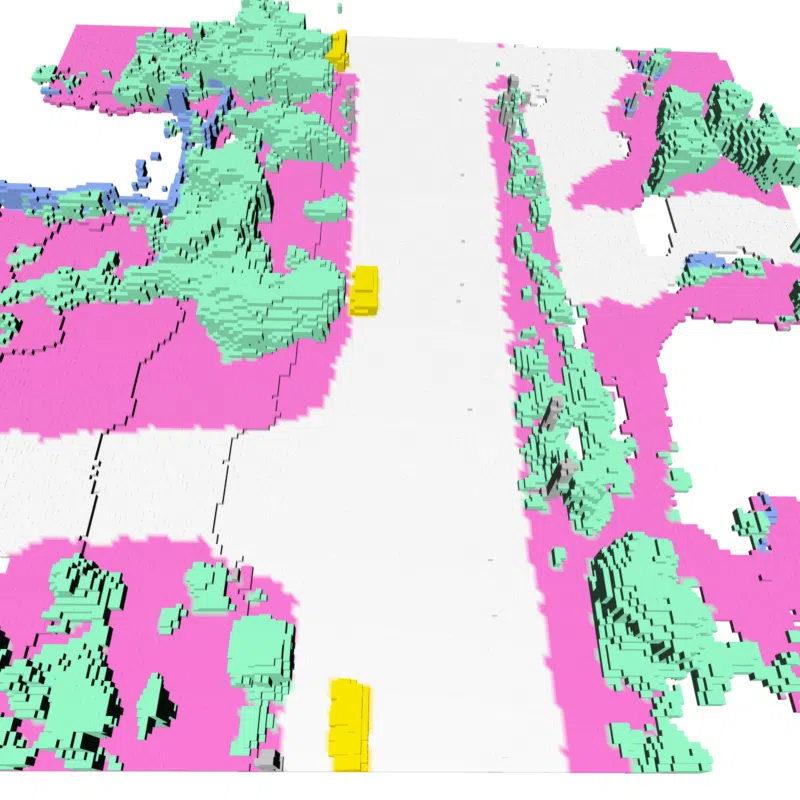
2. HexPlane Conditional Generation
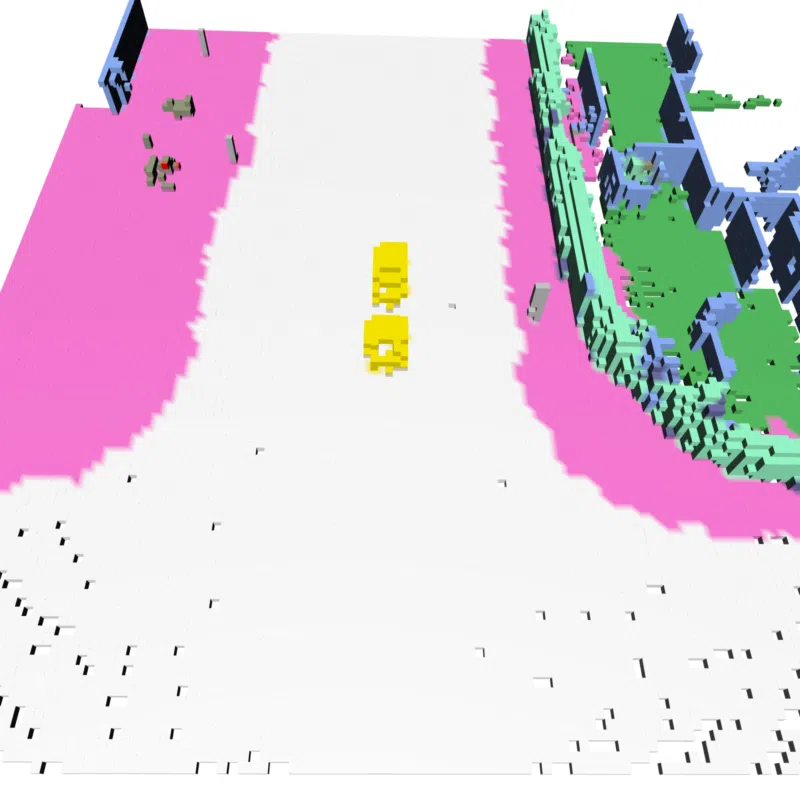
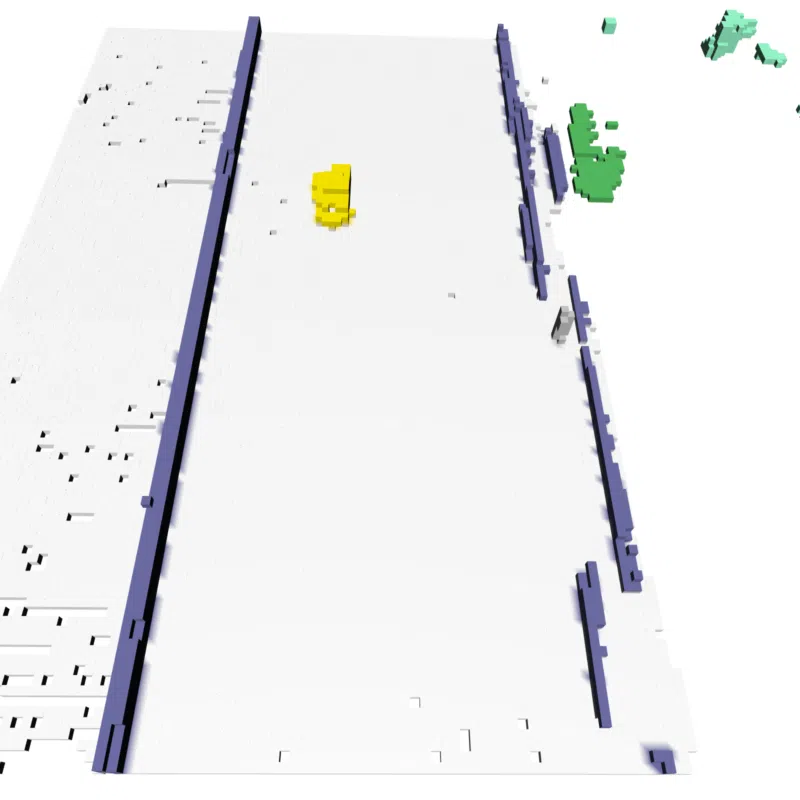
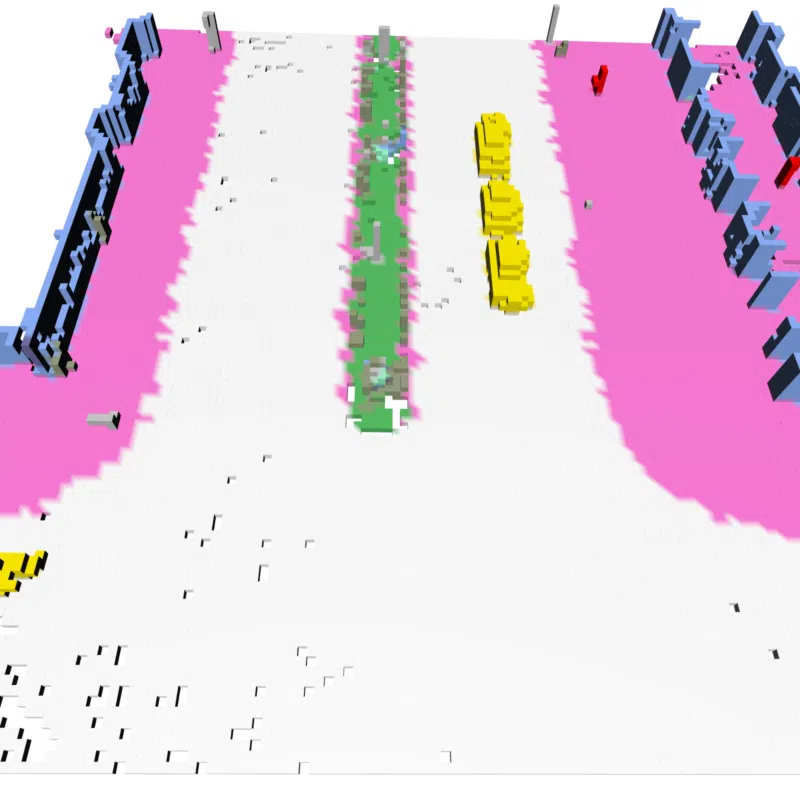
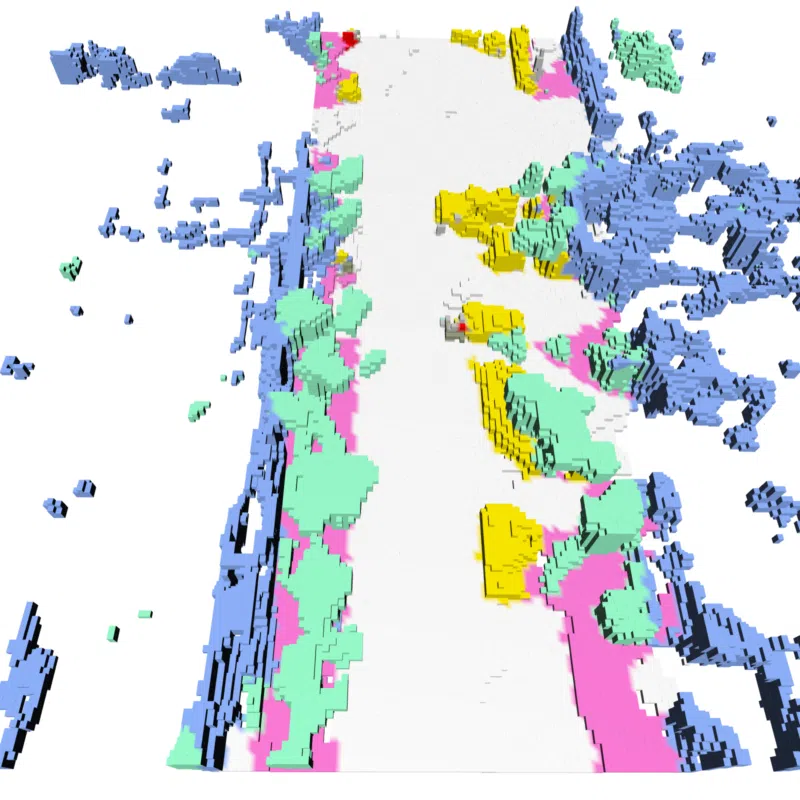
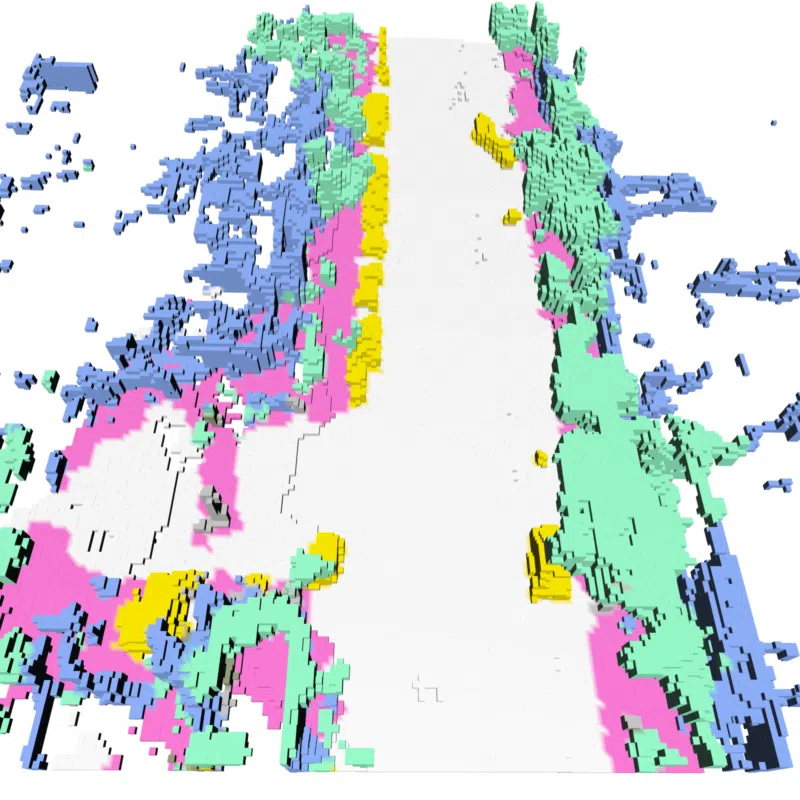
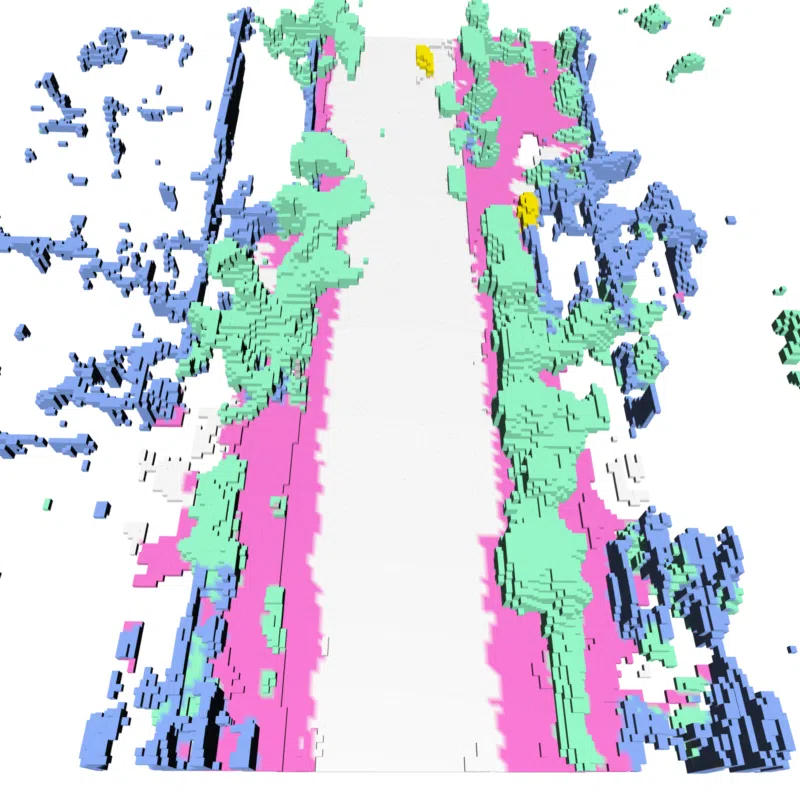
3. Command/Trajectory-Driven Generation
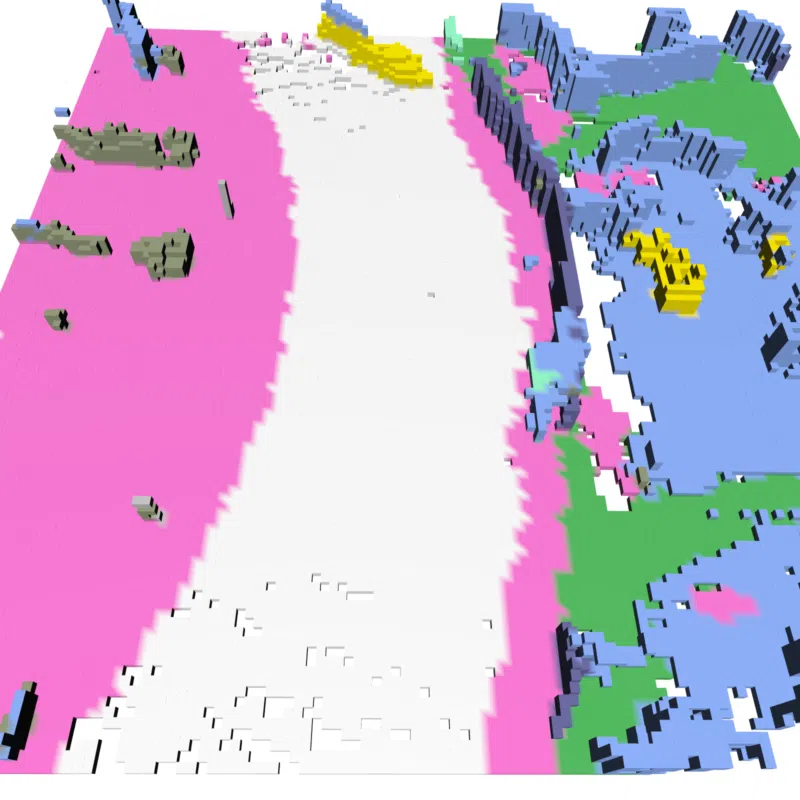
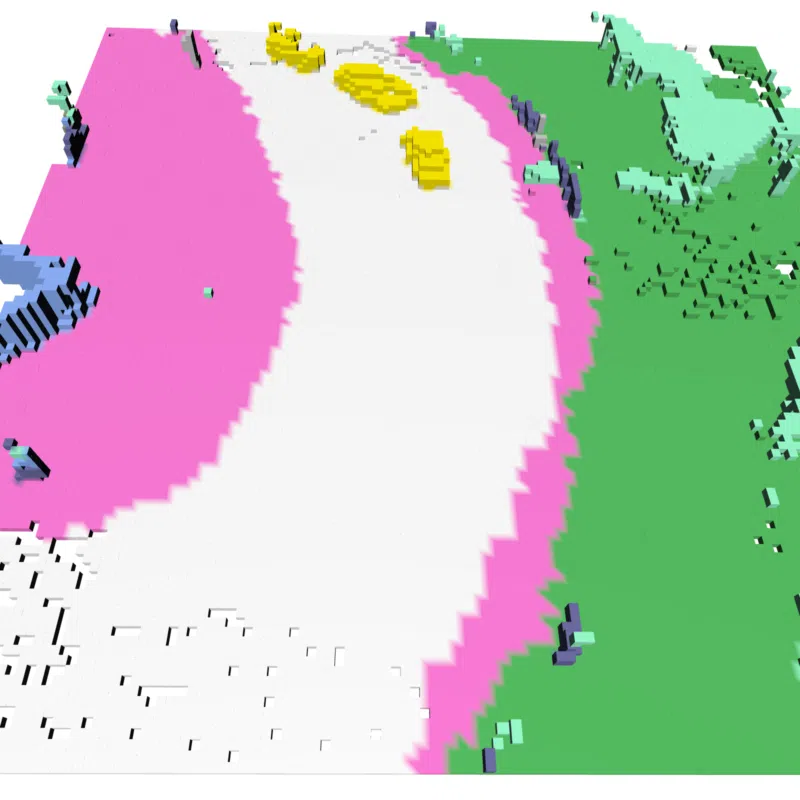
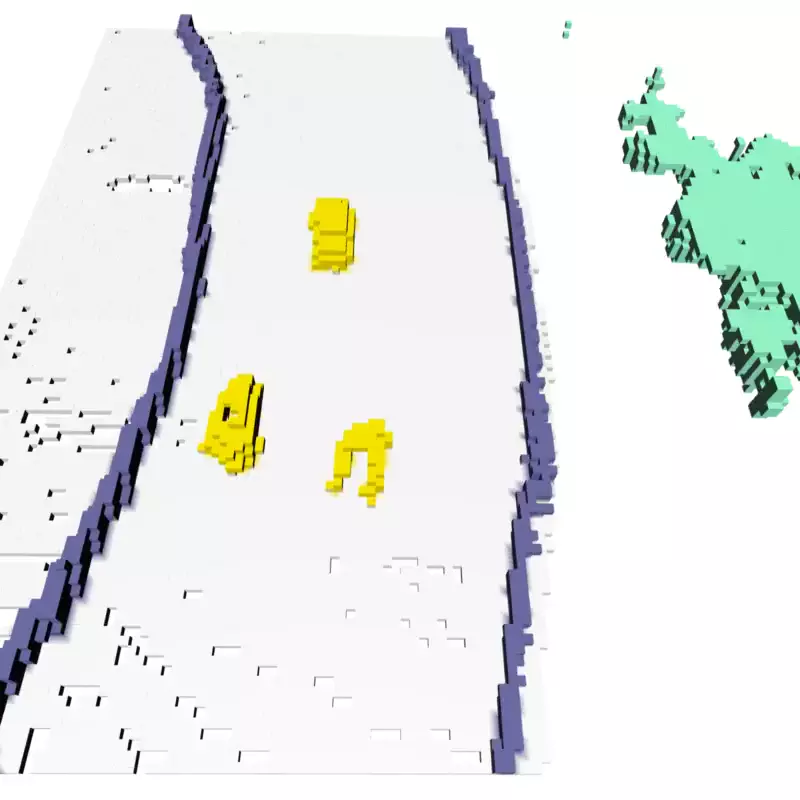
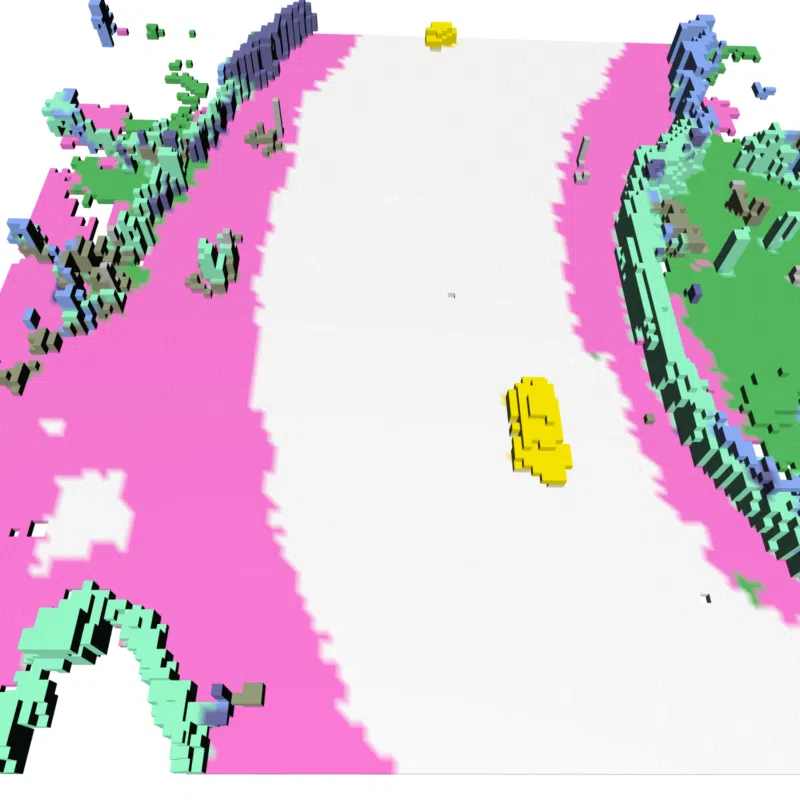
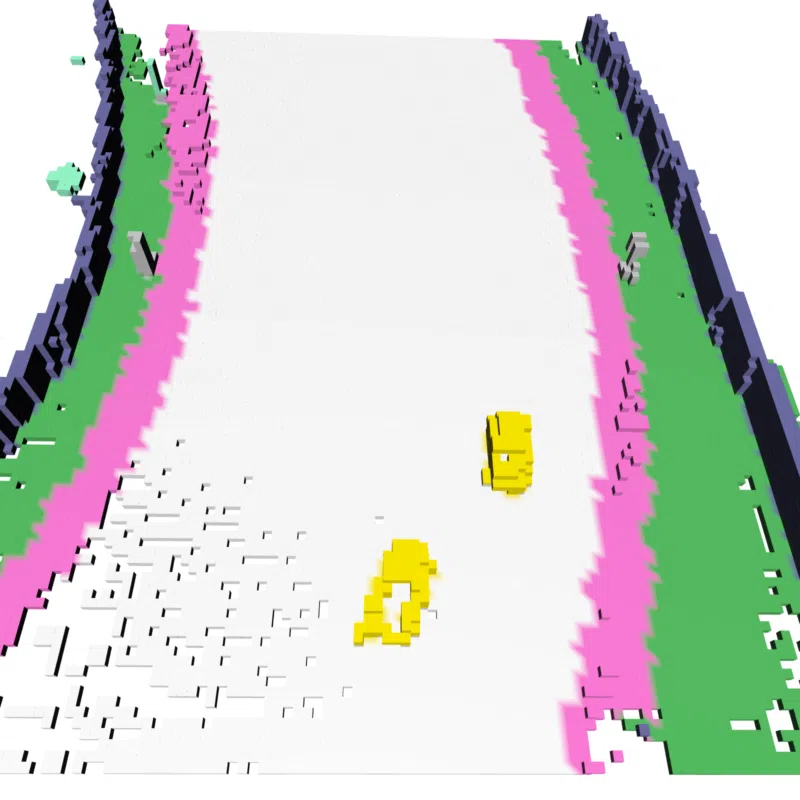
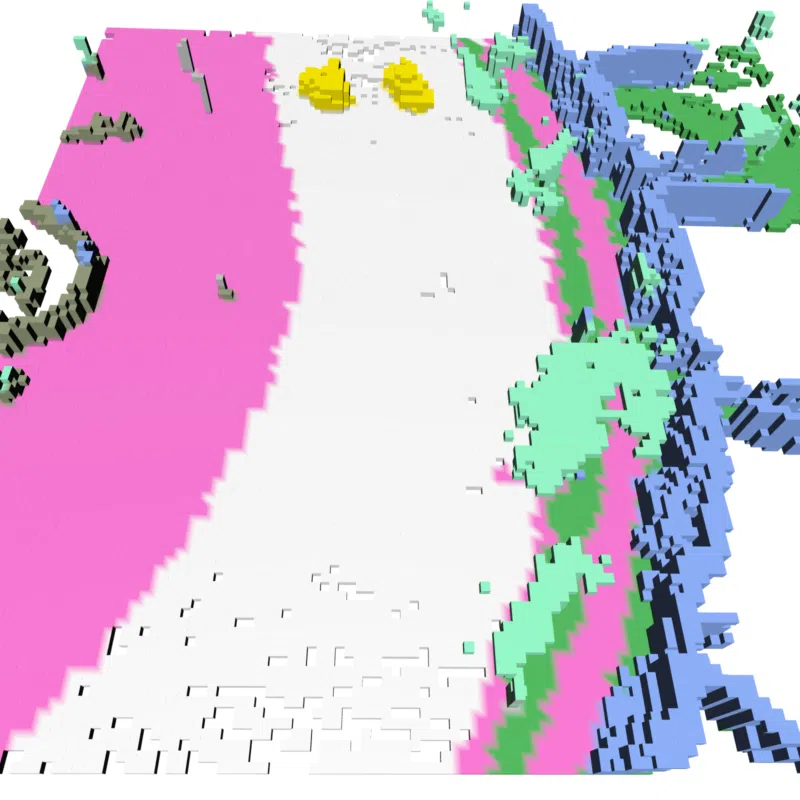
4. Layout-Conditioned Generation
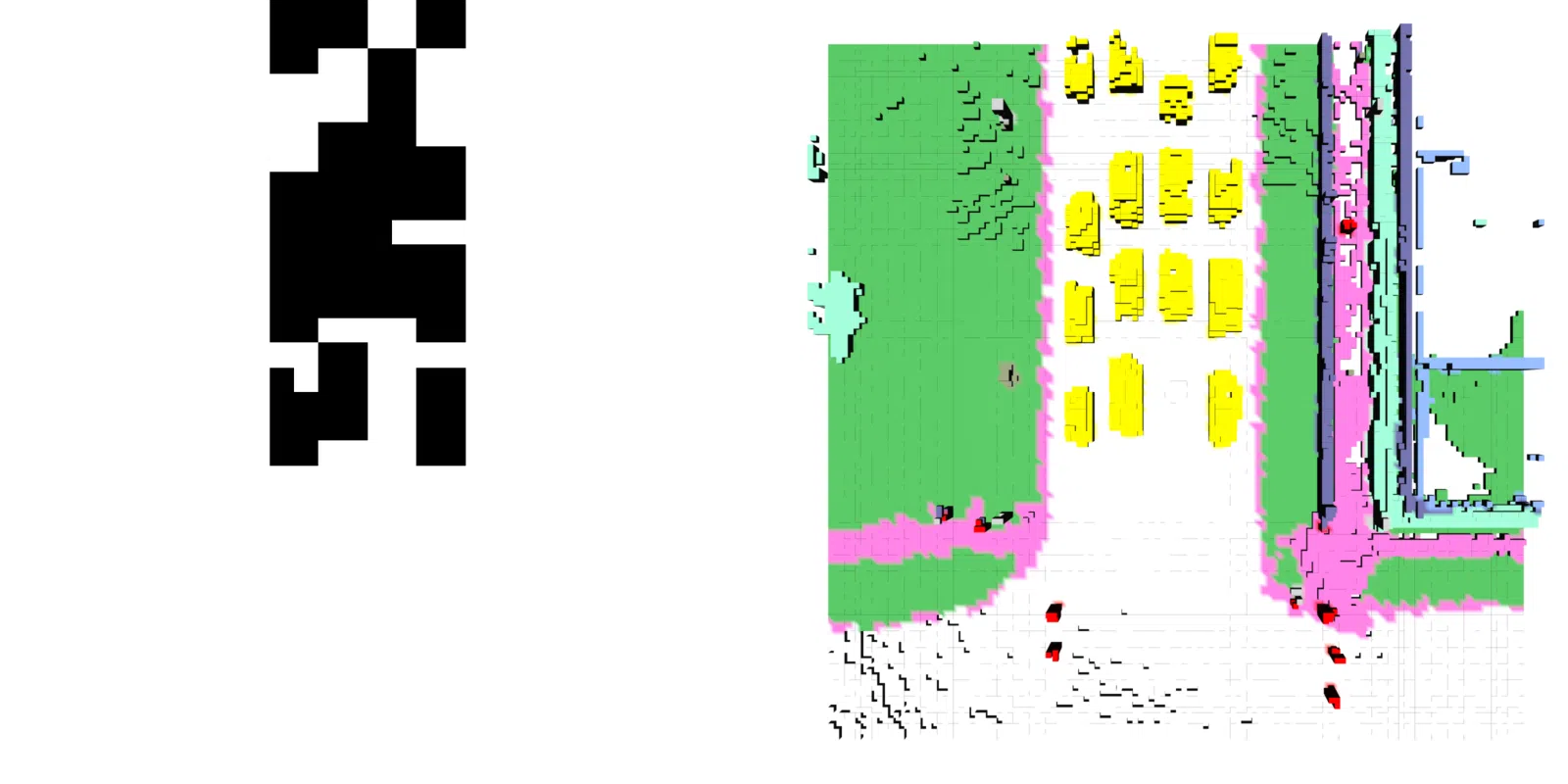
Layout condition
Result
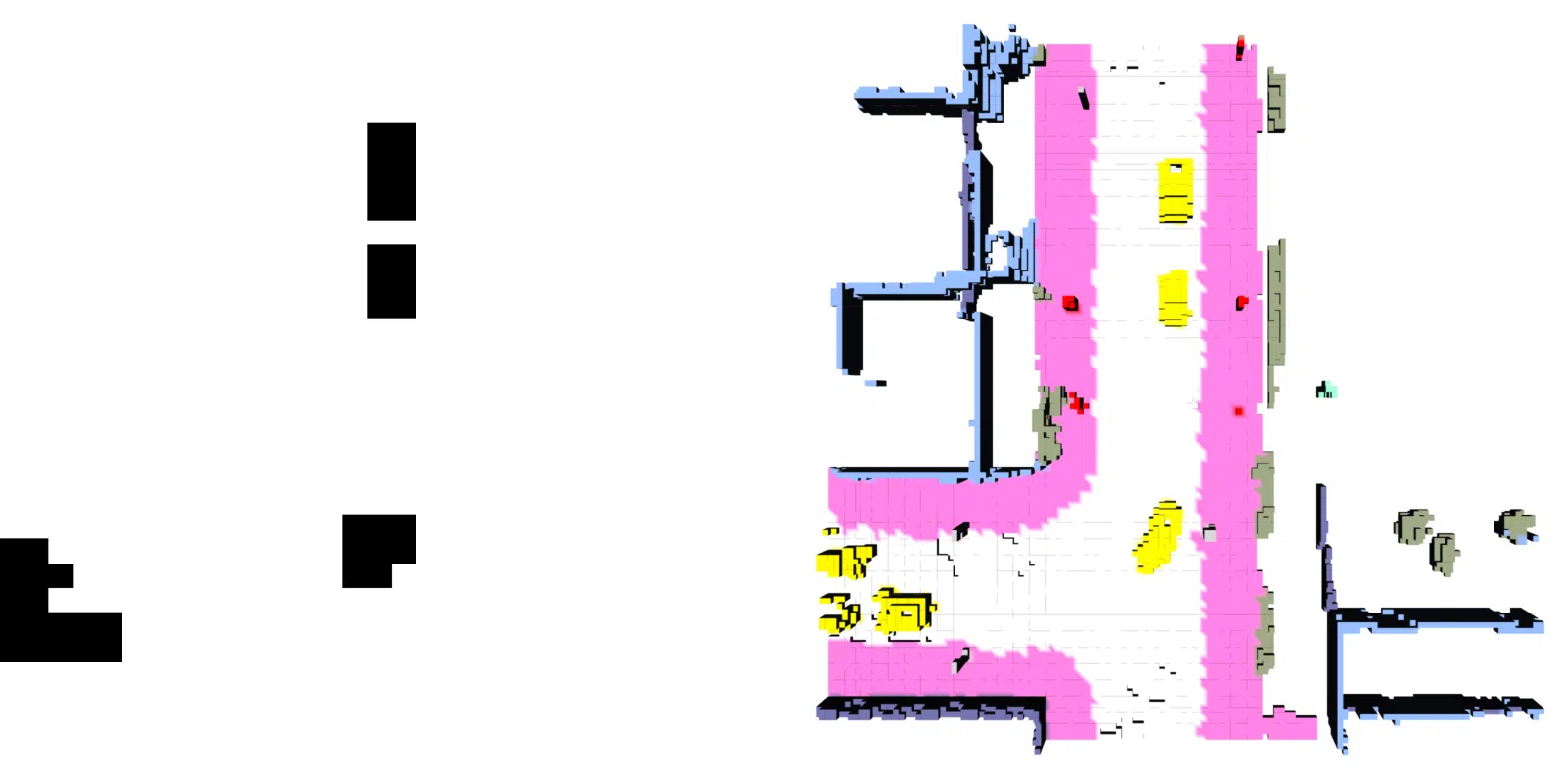
Layout condition
Result
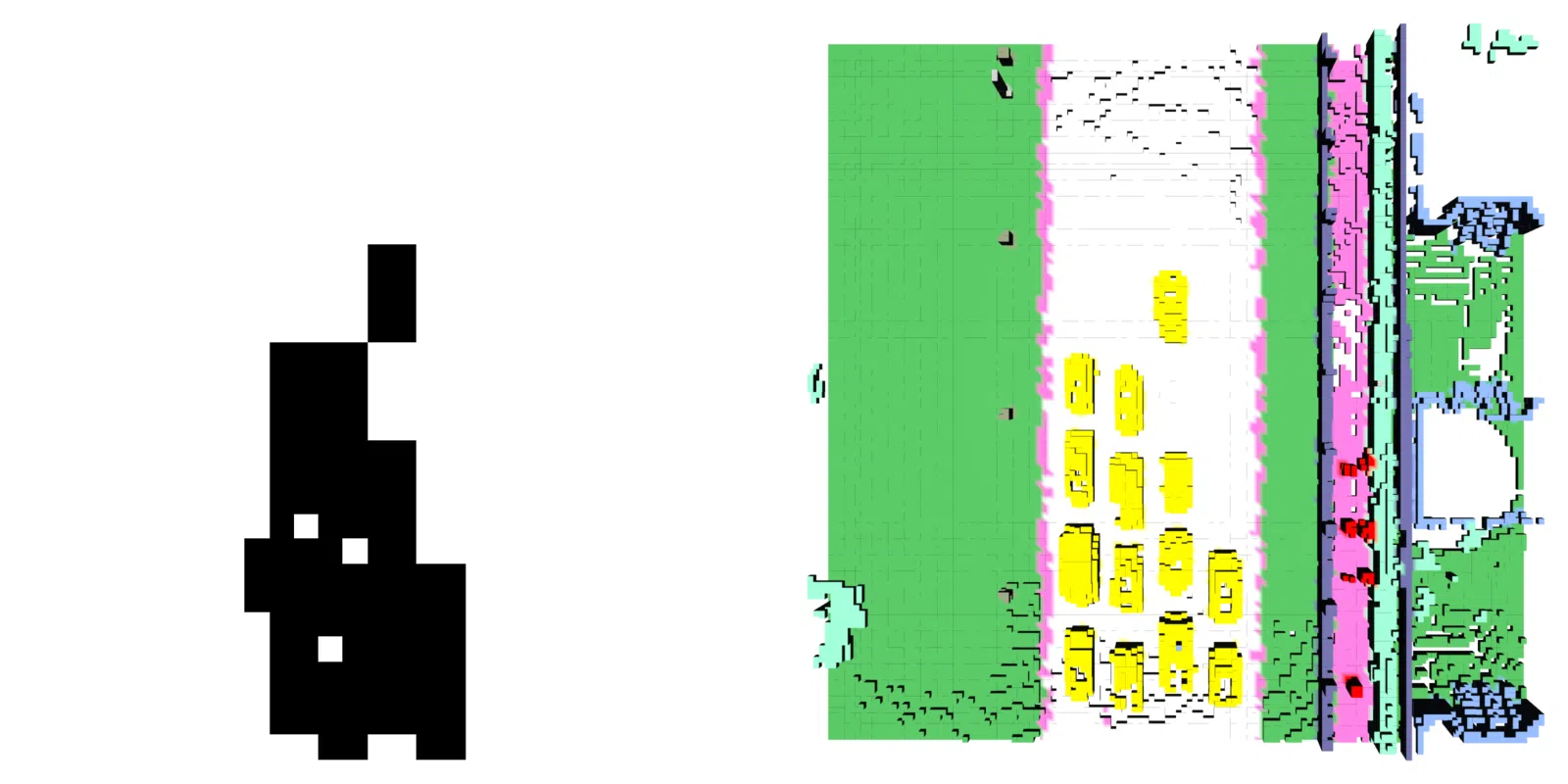
Layout condition
Result
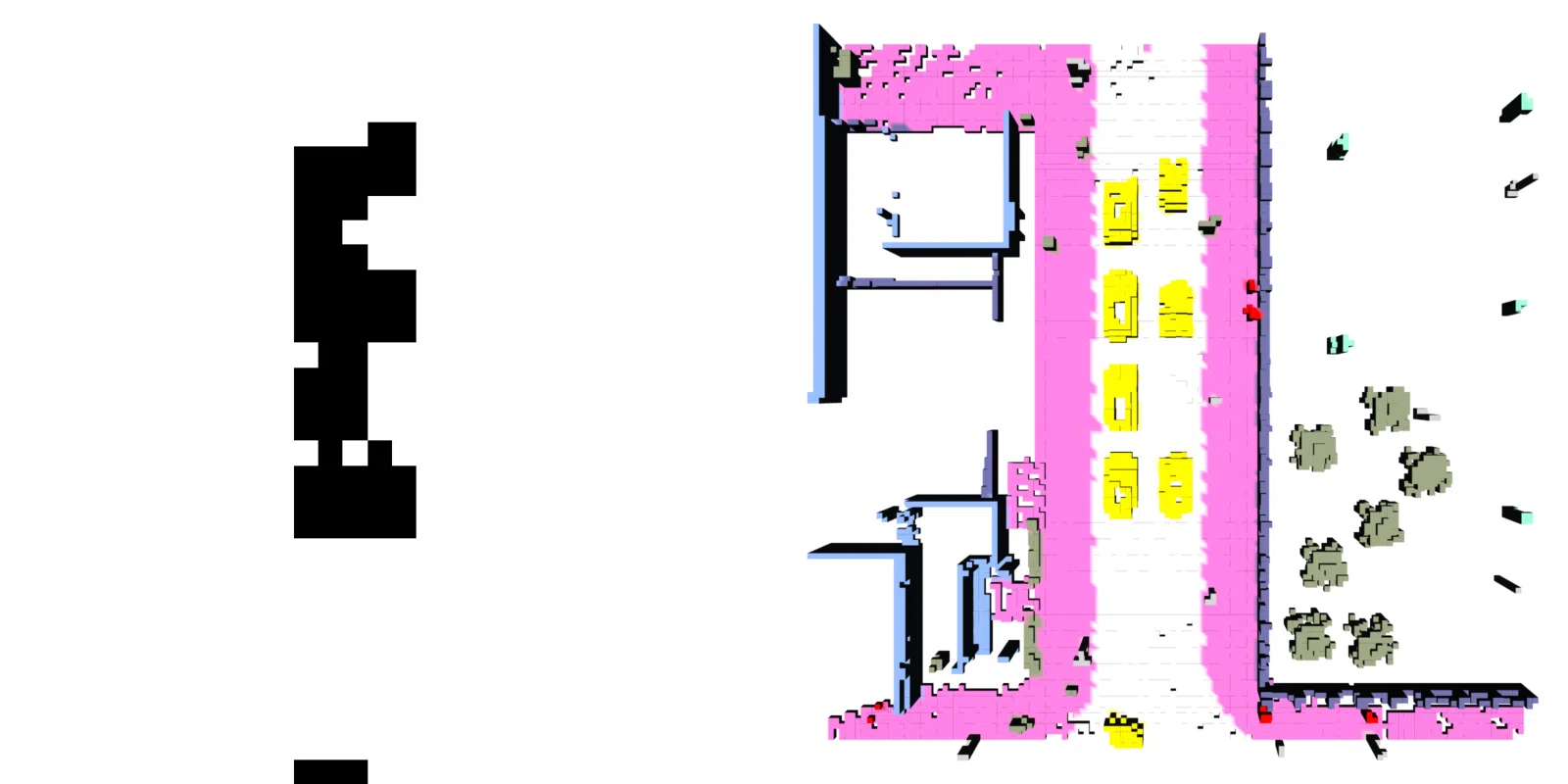
Layout condition
Result
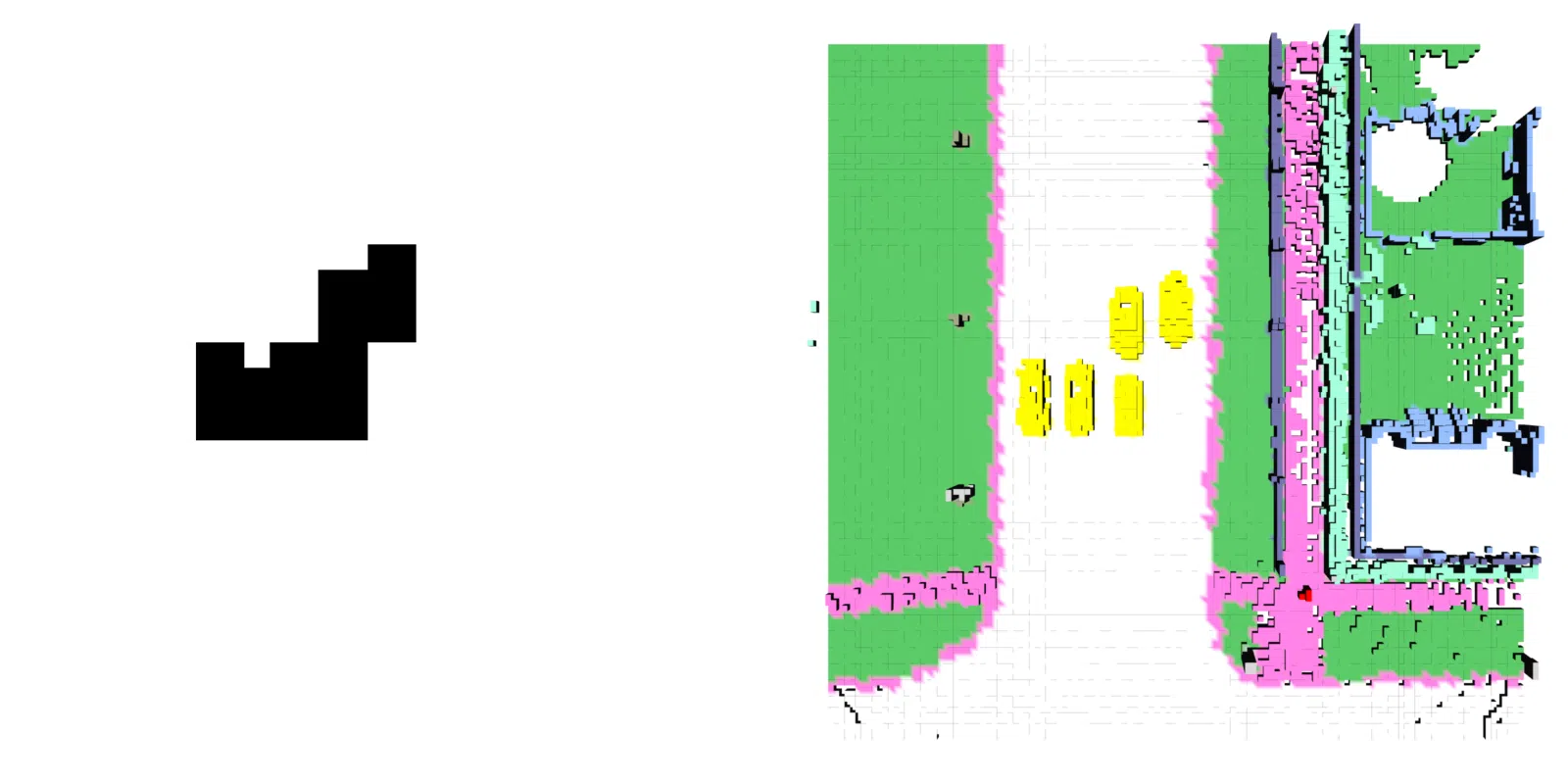
Layout condition
Result
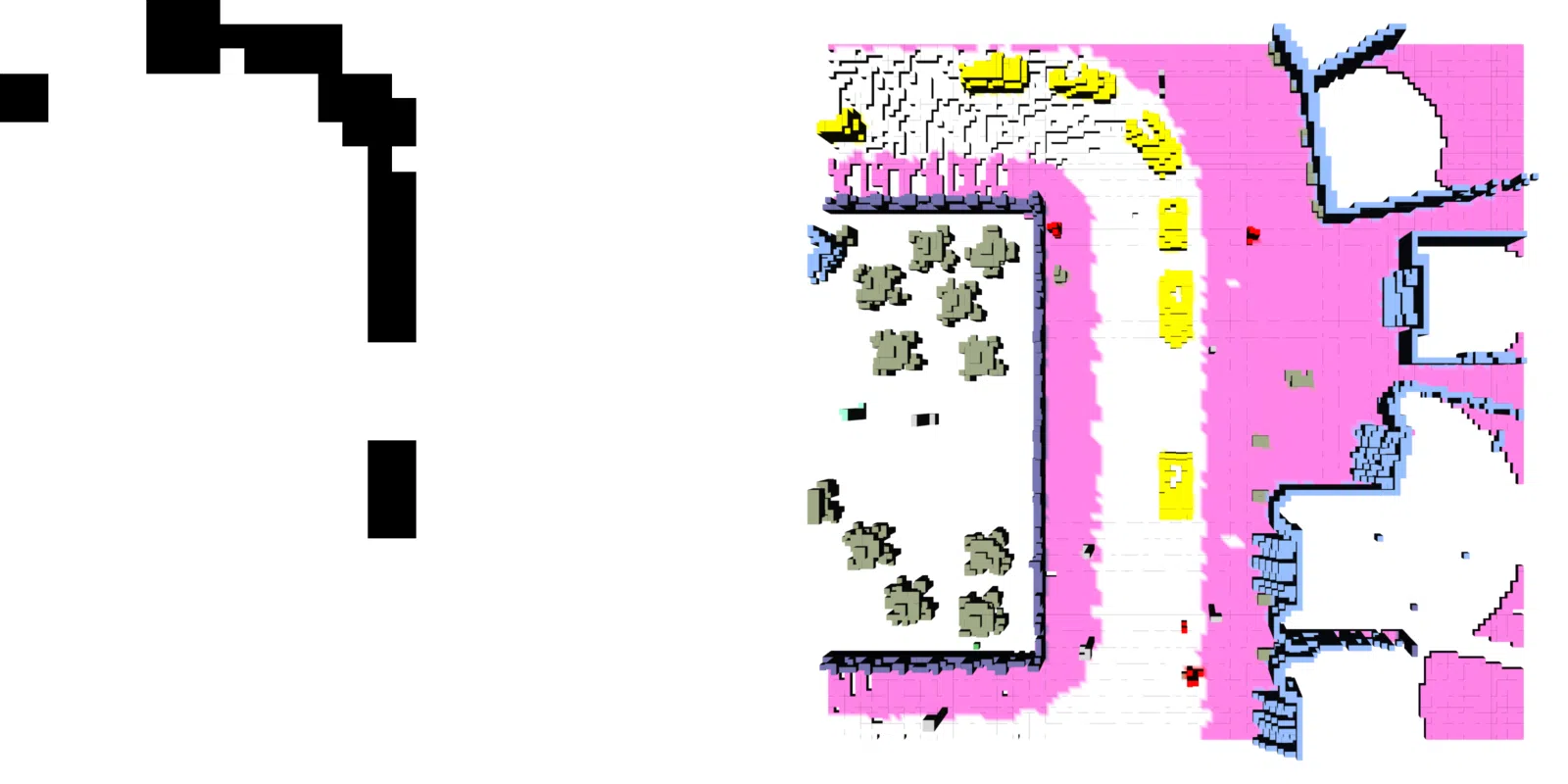
Layout condition
Result
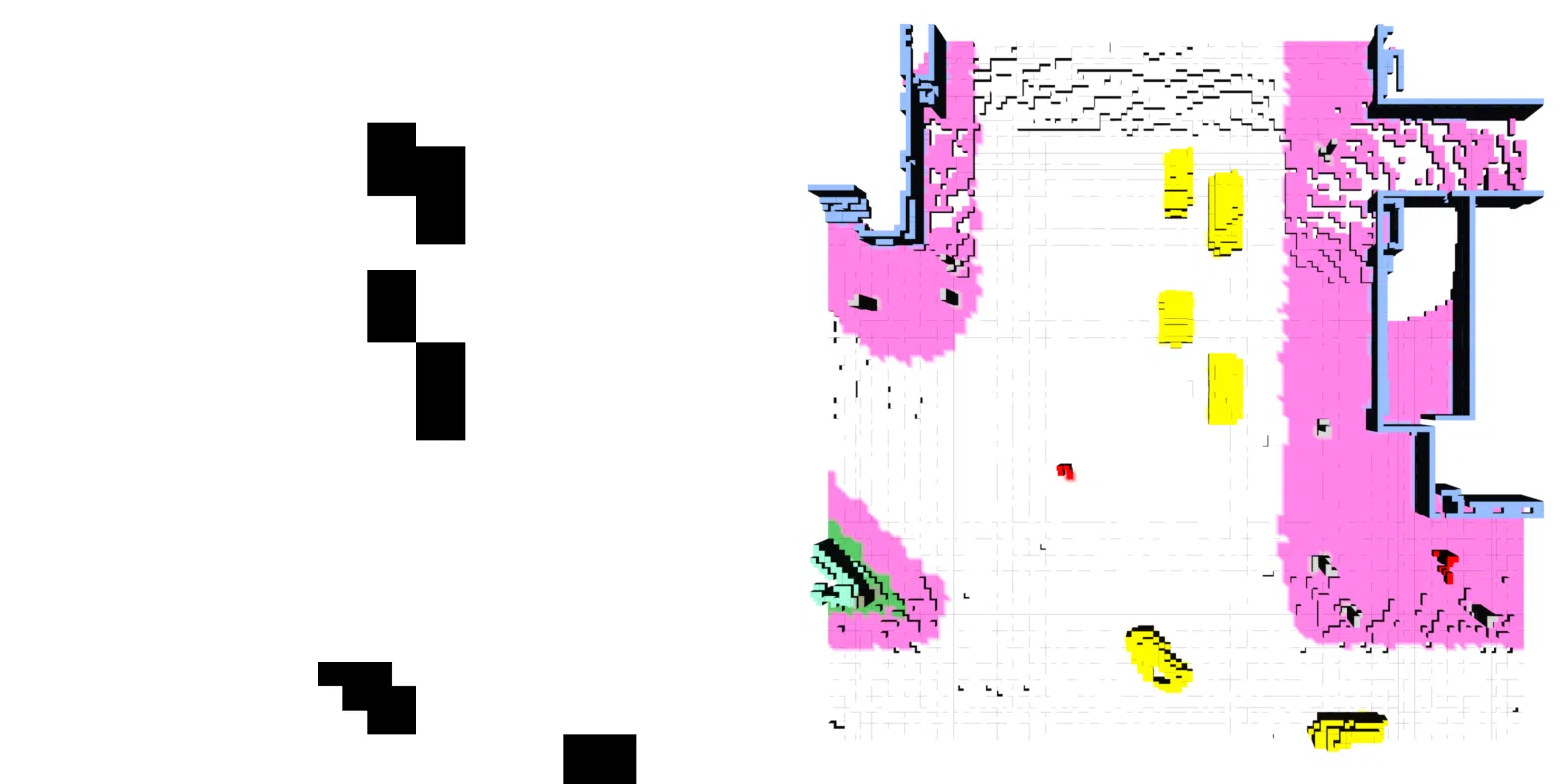
Layout condition
Result
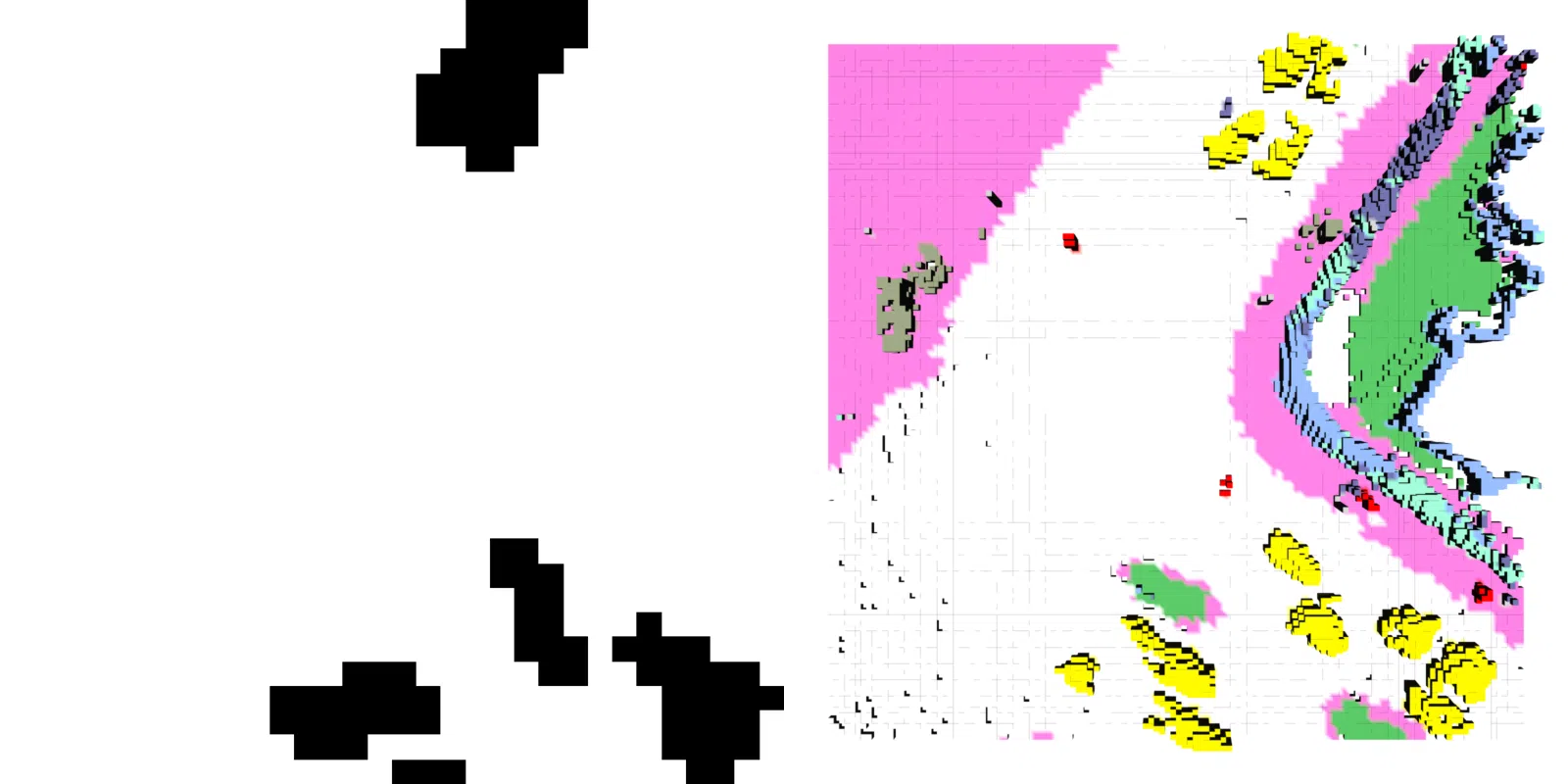
Layout condition
Result
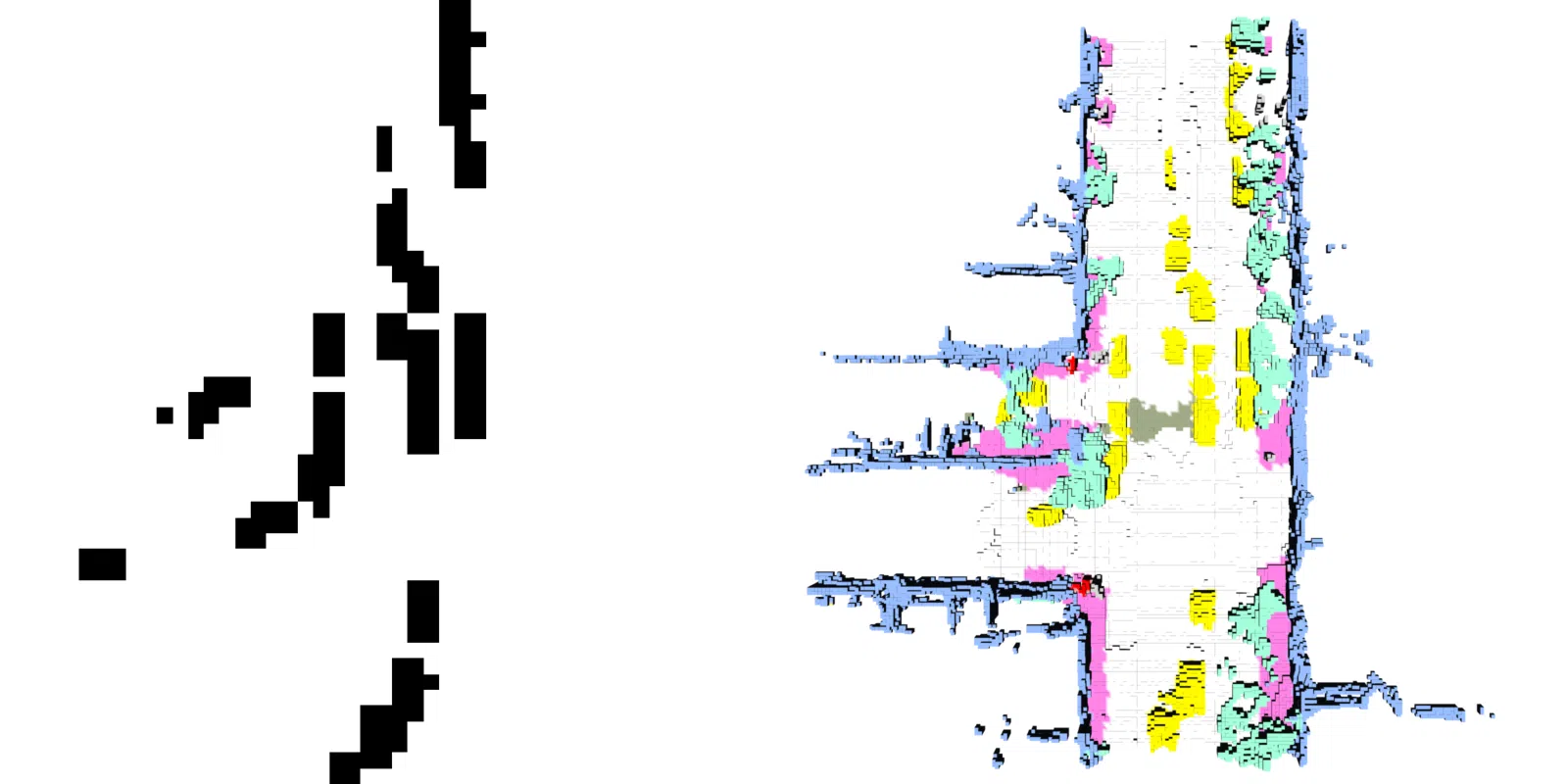
Layout condition
Result
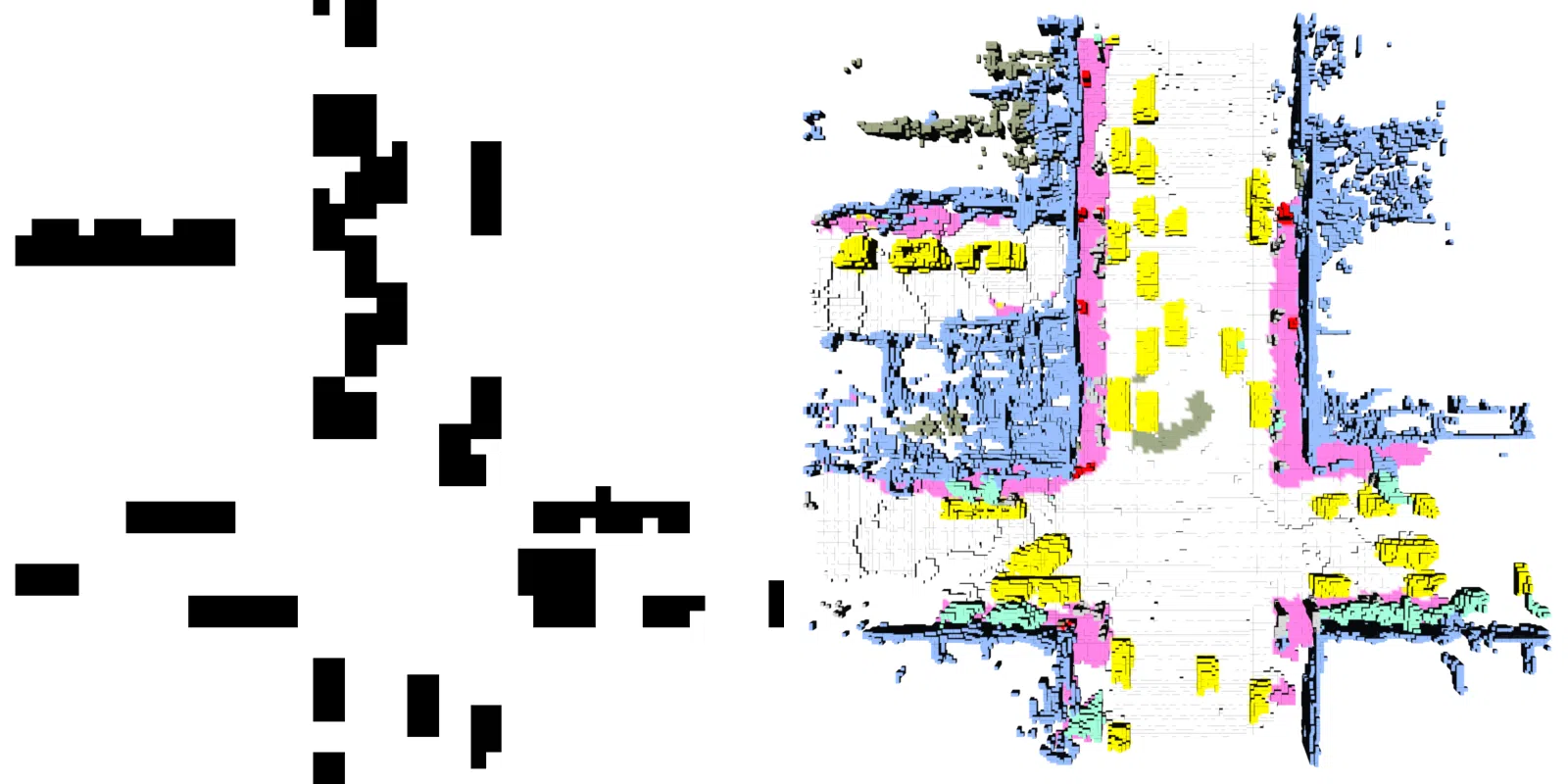
Layout condition
Result
5. Dynamic Scene Inpainting
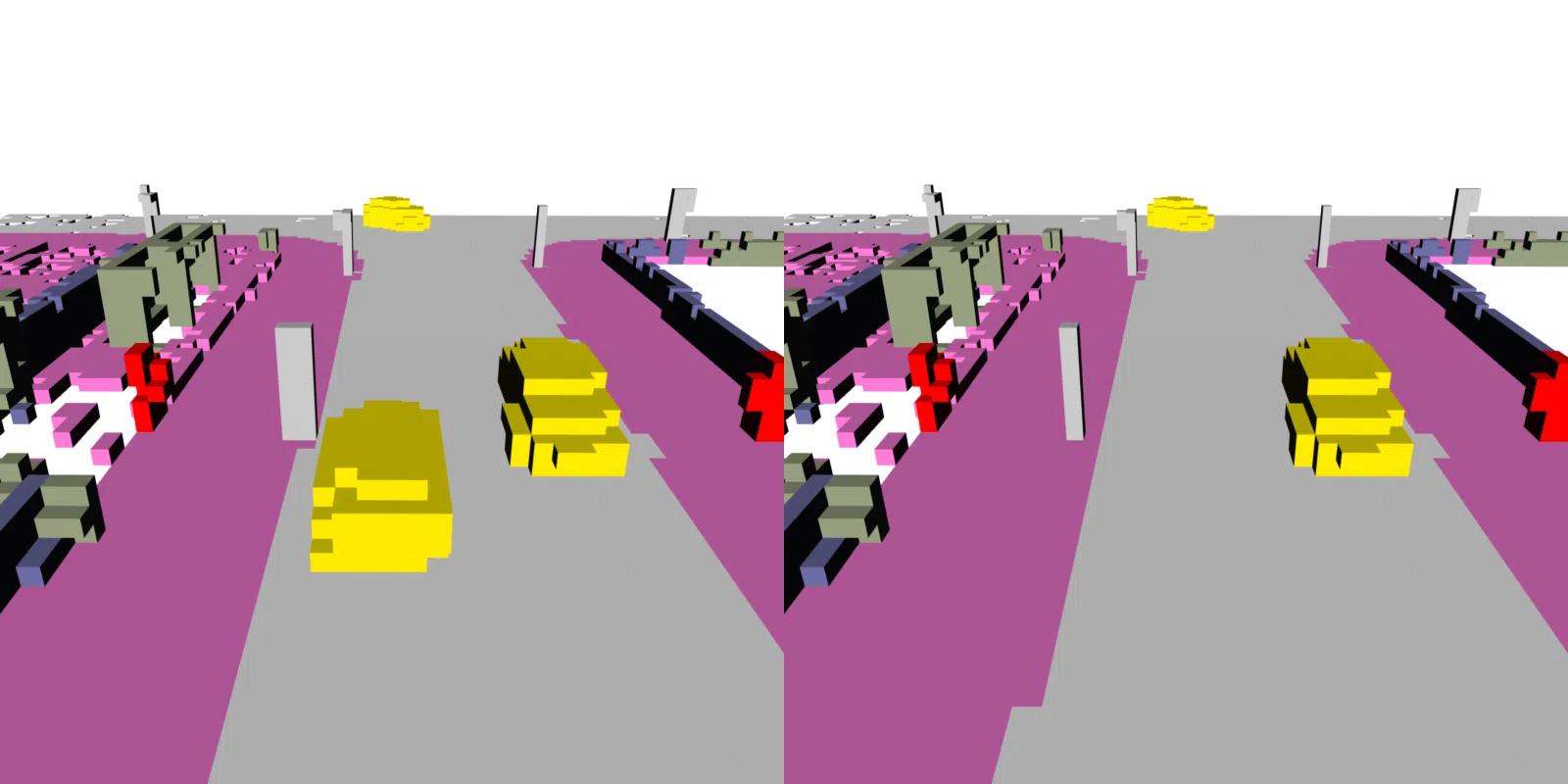
Before inpainting
After inpainting
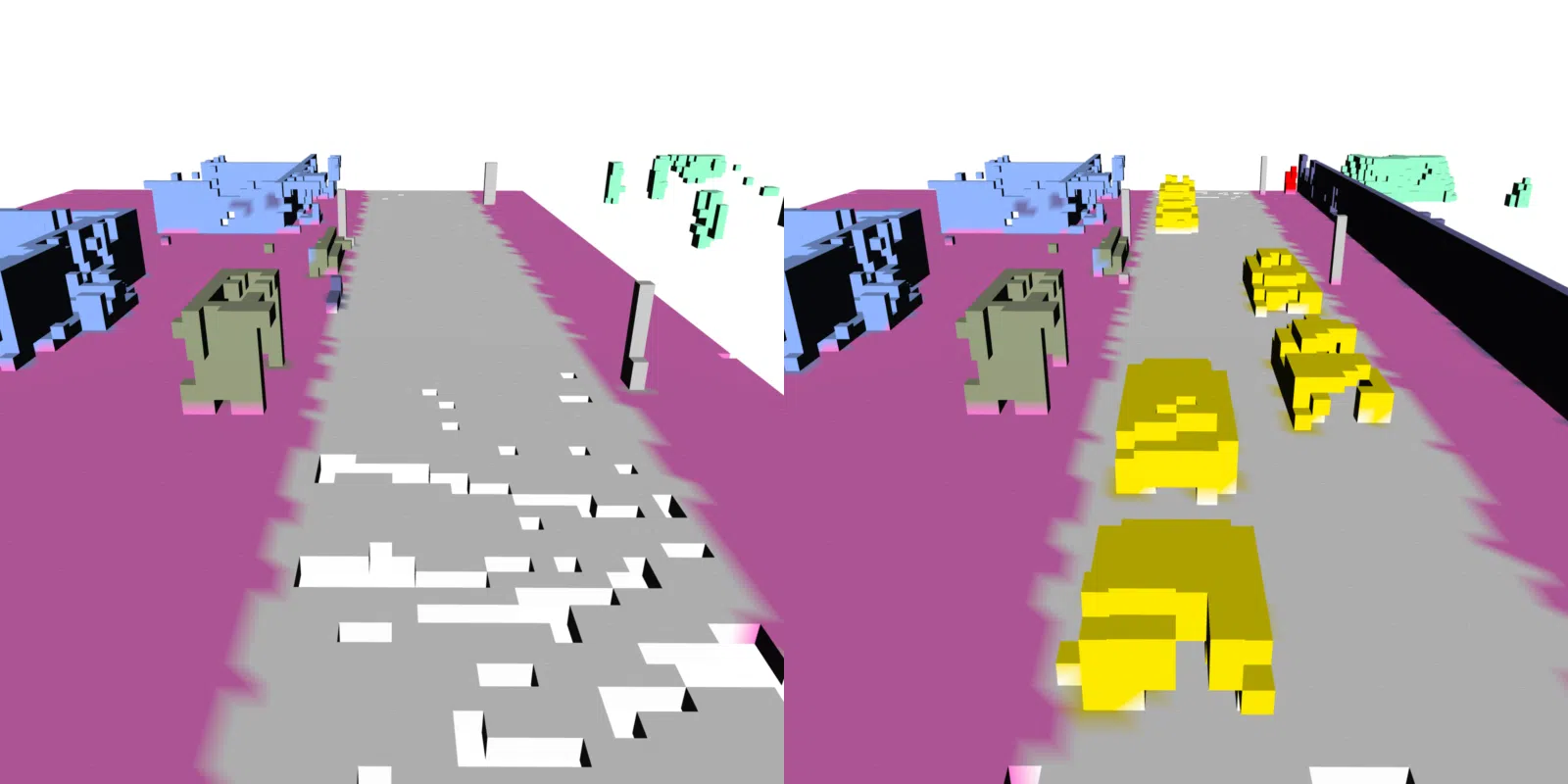
Before inpainting
After inpainting
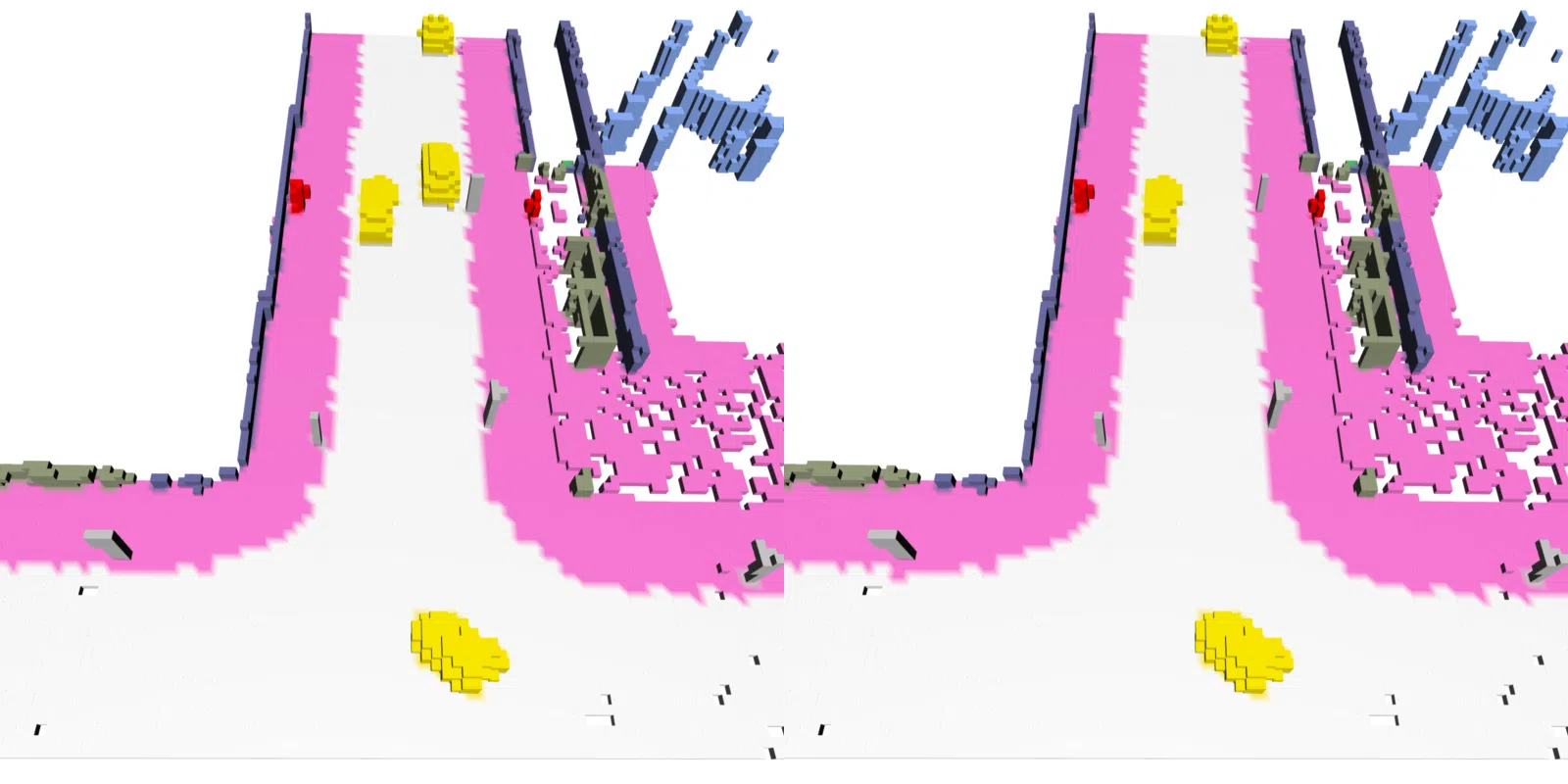
Before inpainting
After inpainting
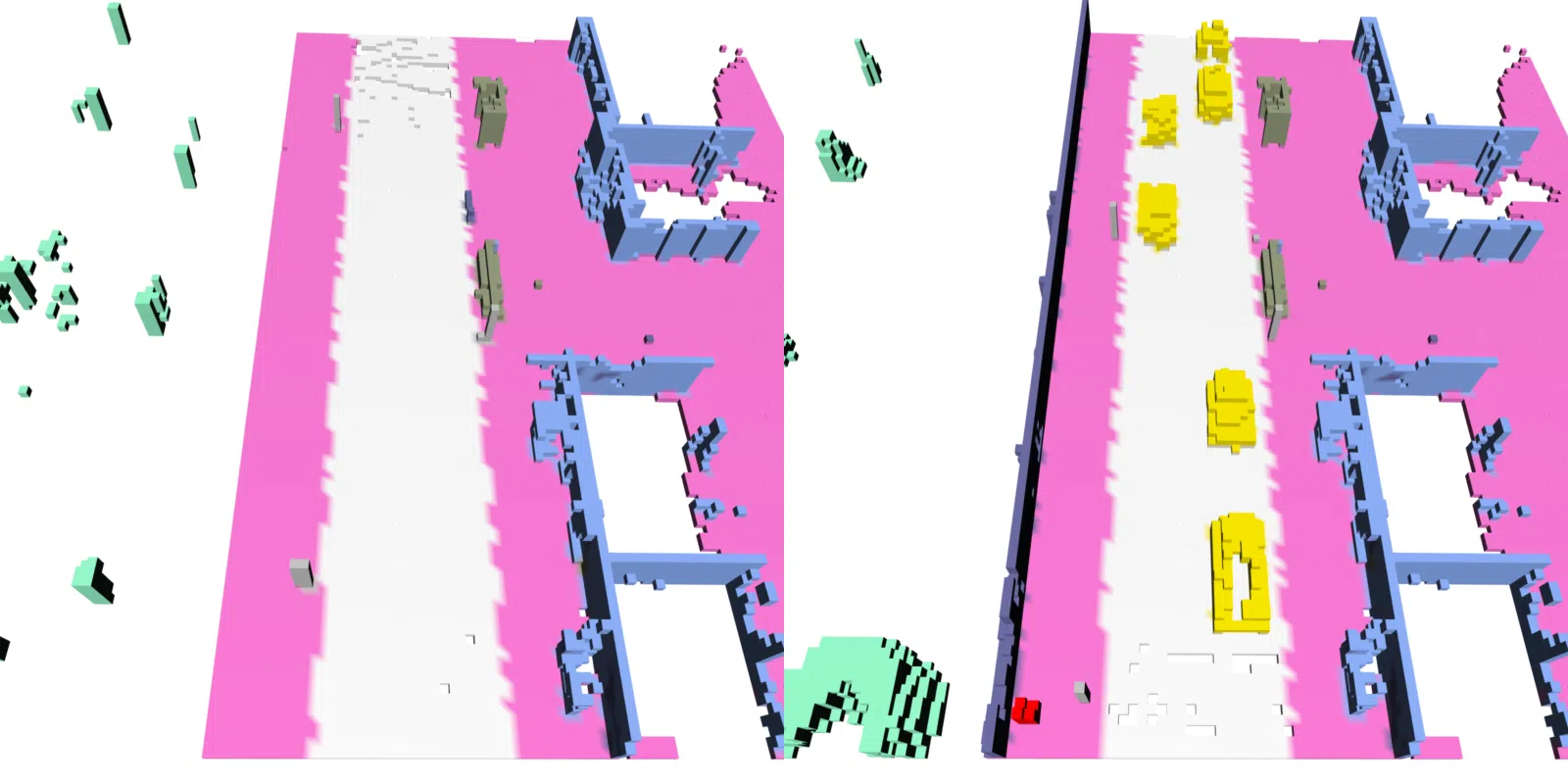
Before inpainting
After inpainting
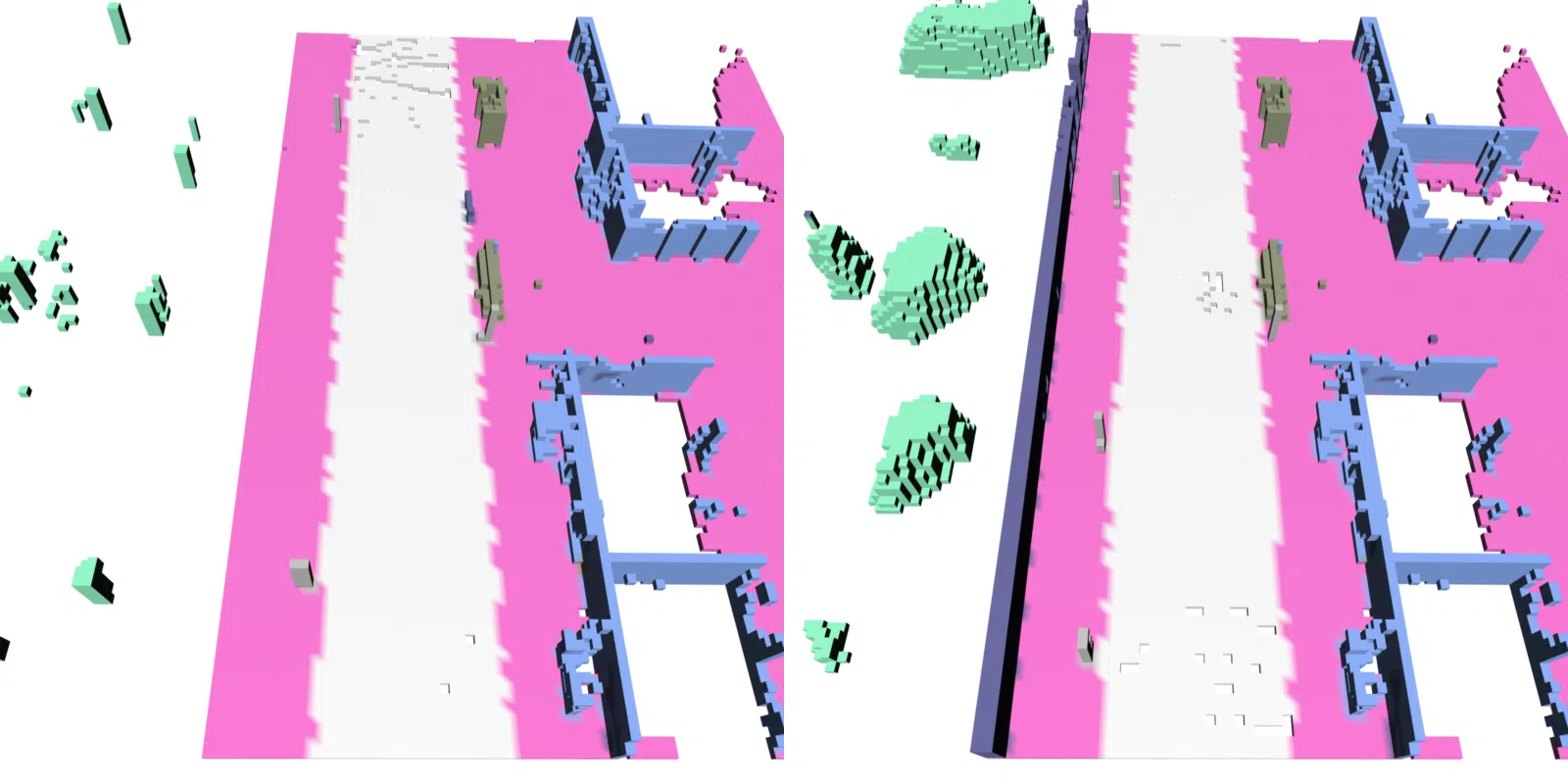
Before inpainting
After inpainting
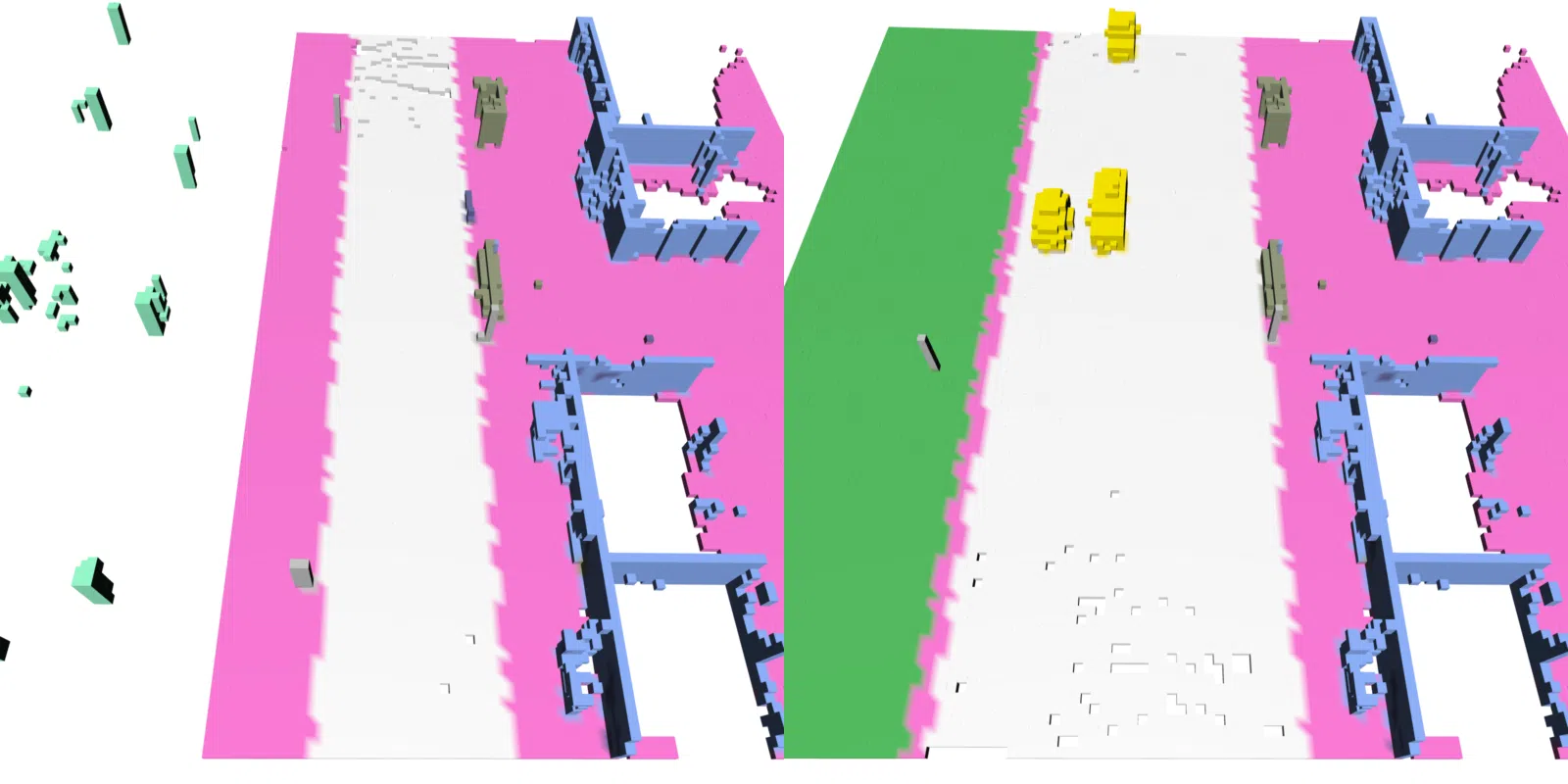
Before inpainting
After inpainting
6. Dynamic Scene Outpainting
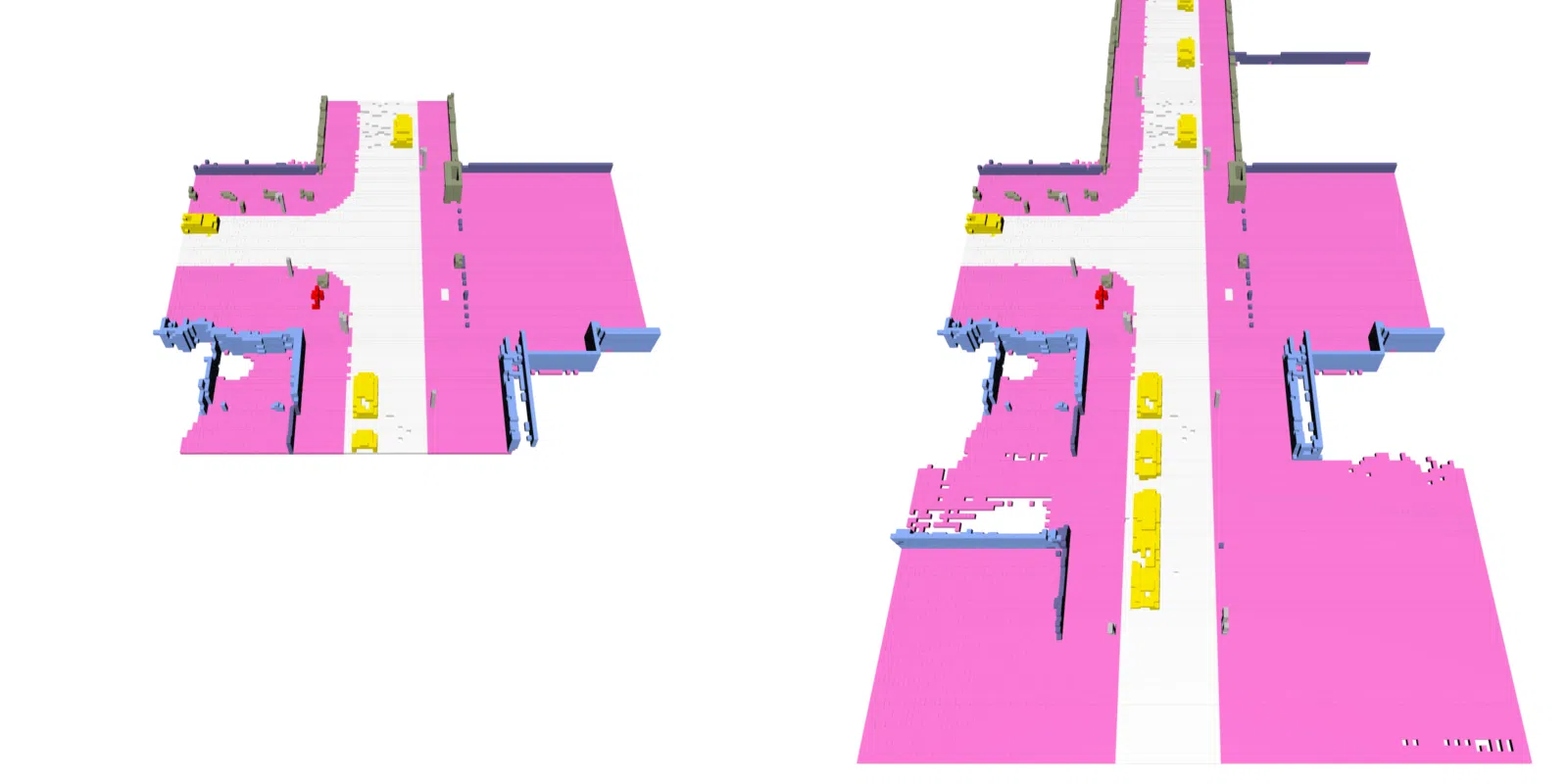
Before outpainting
After outpainting
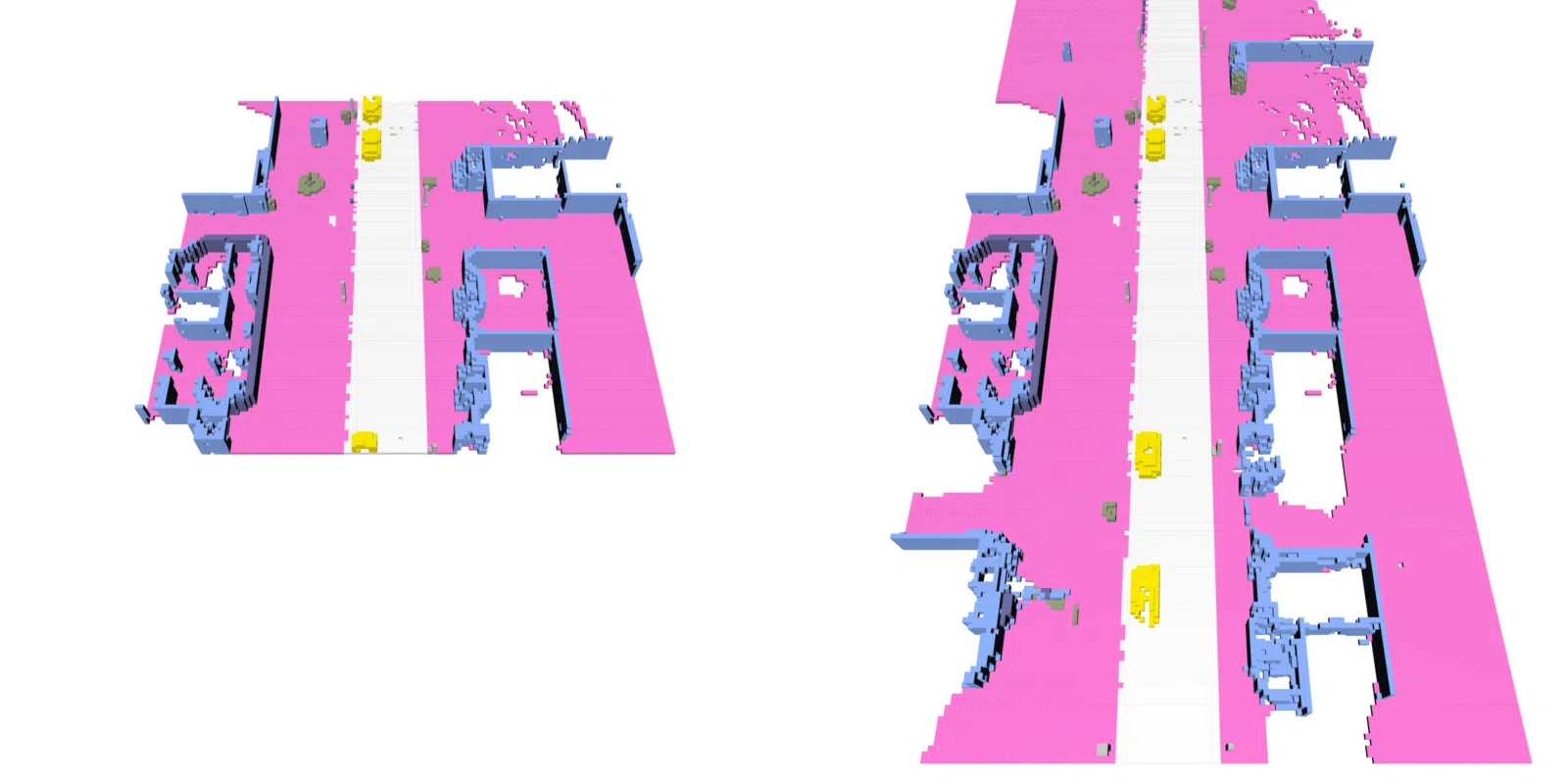
Before outpainting
After outpainting
7. Single Occupancy Conditional Generation
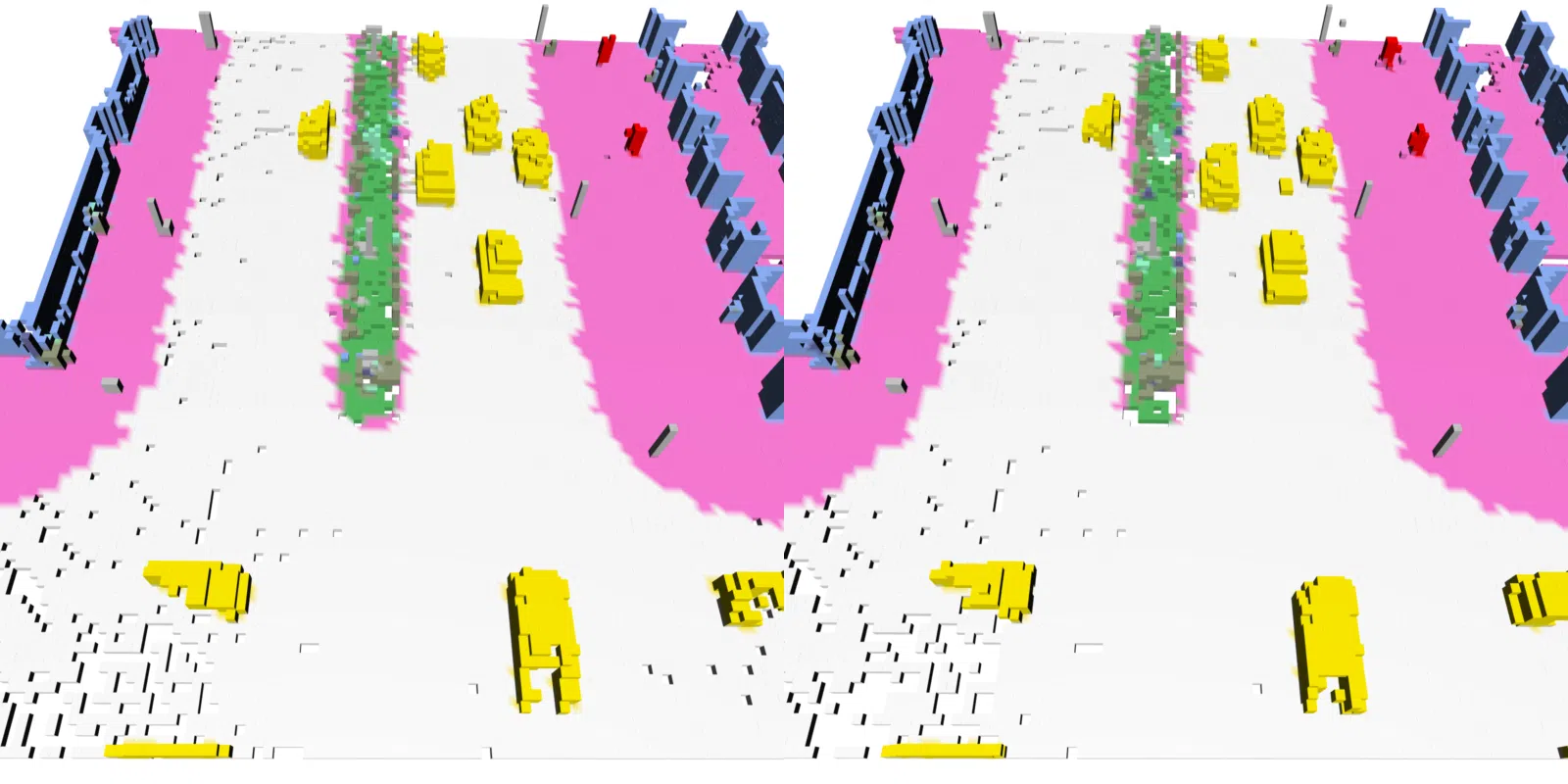
Occupancy condition
Result
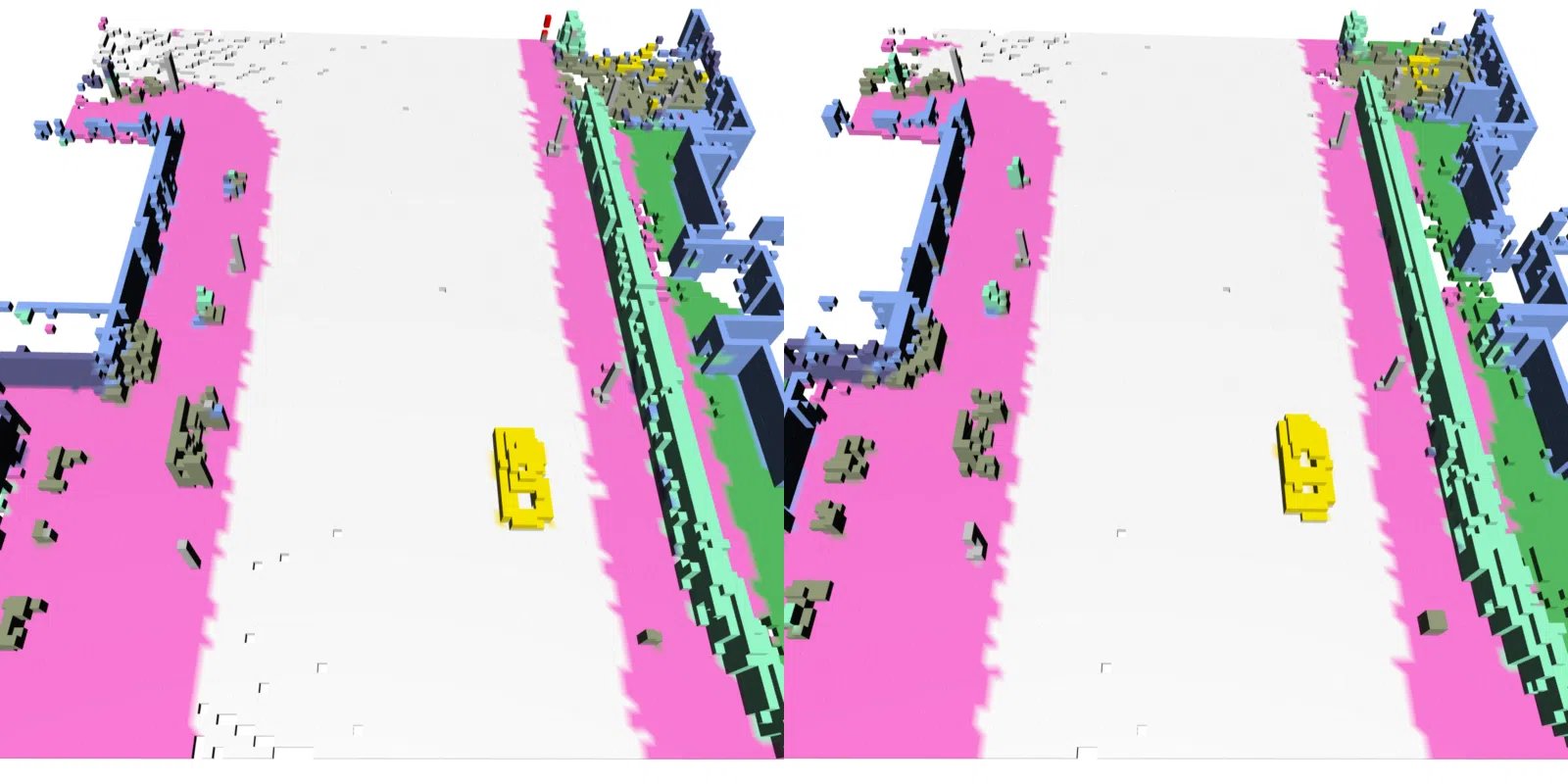
Occupancy condition
Result
BibTeX
@inproceedings{bian2025dynamiccity,
title={DynamicCity: Large-Scale 4D Occupancy Generation from Dynamic Scenes},
author={Bian, Hengwei and Kong, Lingdong and Xie, Haozhe and Pan, Liang and Qiao, Yu and Liu, Ziwei},
booktitle={Proceedings of the International Conference on Learning Representations (ICLR)},
year={2025},
}